Merge branch 'google:master' into image-classification-python
This commit is contained in:
commit
f2e42d16bd
|
@ -51,6 +51,7 @@ RUN update-alternatives --install /usr/bin/gcc gcc /usr/bin/gcc-8 100 --slave /u
|
|||
RUN pip3 install --upgrade setuptools
|
||||
RUN pip3 install wheel
|
||||
RUN pip3 install future
|
||||
RUN pip3 install absl-py numpy opencv-contrib-python protobuf==3.20.1
|
||||
RUN pip3 install six==1.14.0
|
||||
RUN pip3 install tensorflow==2.2.0
|
||||
RUN pip3 install tf_slim
|
||||
|
|
|
@ -4,7 +4,7 @@ title: Home
|
|||
nav_order: 1
|
||||
---
|
||||
|
||||

|
||||

|
||||
|
||||
--------------------------------------------------------------------------------
|
||||
|
||||
|
@ -13,21 +13,21 @@ nav_order: 1
|
|||
[MediaPipe](https://google.github.io/mediapipe/) offers cross-platform, customizable
|
||||
ML solutions for live and streaming media.
|
||||
|
||||
 | 
|
||||
 | 
|
||||
:------------------------------------------------------------------------------------------------------------: | :----------------------------------------------------:
|
||||
***End-to-End acceleration***: *Built-in fast ML inference and processing accelerated even on common hardware* | ***Build once, deploy anywhere***: *Unified solution works across Android, iOS, desktop/cloud, web and IoT*
|
||||
 | 
|
||||
 | 
|
||||
***Ready-to-use solutions***: *Cutting-edge ML solutions demonstrating full power of the framework* | ***Free and open source***: *Framework and solutions both under Apache 2.0, fully extensible and customizable*
|
||||
|
||||
## ML solutions in MediaPipe
|
||||
|
||||
Face Detection | Face Mesh | Iris | Hands | Pose | Holistic
|
||||
:----------------------------------------------------------------------------------------------------------------------------: | :-------------------------------------------------------------------------------------------------------------: | :-------------------------------------------------------------------------------------------------------: | :--------------------------------------------------------------------------------------------------------: | :-------------------------------------------------------------------------------------------------------: | :------:
|
||||
[](https://google.github.io/mediapipe/solutions/face_detection) | [](https://google.github.io/mediapipe/solutions/face_mesh) | [](https://google.github.io/mediapipe/solutions/iris) | [](https://google.github.io/mediapipe/solutions/hands) | [](https://google.github.io/mediapipe/solutions/pose) | [](https://google.github.io/mediapipe/solutions/holistic)
|
||||
[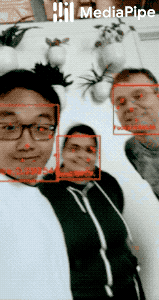](https://google.github.io/mediapipe/solutions/face_detection) | [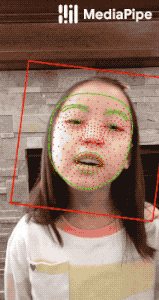](https://google.github.io/mediapipe/solutions/face_mesh) | [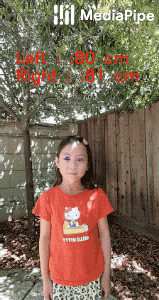](https://google.github.io/mediapipe/solutions/iris) | [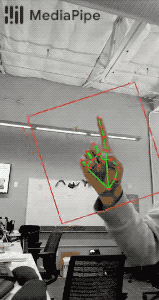](https://google.github.io/mediapipe/solutions/hands) | [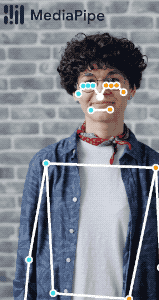](https://google.github.io/mediapipe/solutions/pose) | [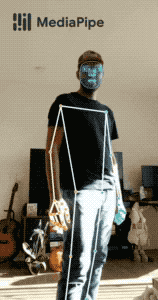](https://google.github.io/mediapipe/solutions/holistic)
|
||||
|
||||
Hair Segmentation | Object Detection | Box Tracking | Instant Motion Tracking | Objectron | KNIFT
|
||||
:-------------------------------------------------------------------------------------------------------------------------------------: | :----------------------------------------------------------------------------------------------------------------------------------: | :-------------------------------------------------------------------------------------------------------------------------: | :---------------------------------------------------------------------------------------------------------------------------------------------------: | :-------------------------------------------------------------------------------------------------------------------: | :---:
|
||||
[](https://google.github.io/mediapipe/solutions/hair_segmentation) | [](https://google.github.io/mediapipe/solutions/object_detection) | [](https://google.github.io/mediapipe/solutions/box_tracking) | [](https://google.github.io/mediapipe/solutions/instant_motion_tracking) | [](https://google.github.io/mediapipe/solutions/objectron) | [](https://google.github.io/mediapipe/solutions/knift)
|
||||
[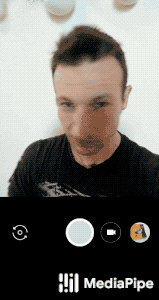](https://google.github.io/mediapipe/solutions/hair_segmentation) | [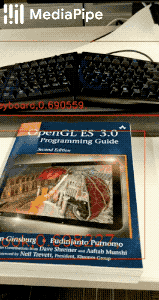](https://google.github.io/mediapipe/solutions/object_detection) | [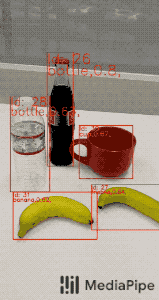](https://google.github.io/mediapipe/solutions/box_tracking) | [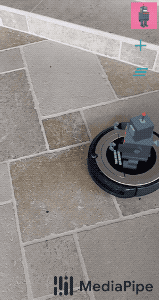](https://google.github.io/mediapipe/solutions/instant_motion_tracking) | [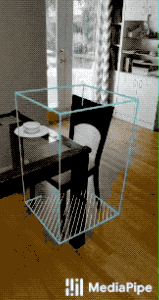](https://google.github.io/mediapipe/solutions/objectron) | [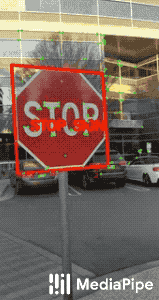](https://google.github.io/mediapipe/solutions/knift)
|
||||
|
||||
<!-- []() in the first cell is needed to preserve table formatting in GitHub Pages. -->
|
||||
<!-- Whenever this table is updated, paste a copy to solutions/solutions.md. -->
|
||||
|
|
|
@ -216,6 +216,50 @@ cc_library(
|
|||
alwayslink = 1,
|
||||
)
|
||||
|
||||
cc_library(
|
||||
name = "inference_runner",
|
||||
hdrs = ["inference_runner.h"],
|
||||
copts = select({
|
||||
# TODO: fix tensor.h not to require this, if possible
|
||||
"//mediapipe:apple": [
|
||||
"-x objective-c++",
|
||||
"-fobjc-arc", # enable reference-counting
|
||||
],
|
||||
"//conditions:default": [],
|
||||
}),
|
||||
visibility = ["//visibility:public"],
|
||||
deps = [
|
||||
"//mediapipe/framework/formats:tensor",
|
||||
"@com_google_absl//absl/status:statusor",
|
||||
],
|
||||
)
|
||||
|
||||
cc_library(
|
||||
name = "inference_interpreter_delegate_runner",
|
||||
srcs = ["inference_interpreter_delegate_runner.cc"],
|
||||
hdrs = ["inference_interpreter_delegate_runner.h"],
|
||||
copts = select({
|
||||
# TODO: fix tensor.h not to require this, if possible
|
||||
"//mediapipe:apple": [
|
||||
"-x objective-c++",
|
||||
"-fobjc-arc", # enable reference-counting
|
||||
],
|
||||
"//conditions:default": [],
|
||||
}),
|
||||
visibility = ["//visibility:public"],
|
||||
deps = [
|
||||
":inference_runner",
|
||||
"//mediapipe/framework/api2:packet",
|
||||
"//mediapipe/framework/formats:tensor",
|
||||
"//mediapipe/framework/port:ret_check",
|
||||
"//mediapipe/util/tflite:tflite_model_loader",
|
||||
"@com_google_absl//absl/status",
|
||||
"@com_google_absl//absl/status:statusor",
|
||||
"@org_tensorflow//tensorflow/lite:framework_stable",
|
||||
"@org_tensorflow//tensorflow/lite/core/api:op_resolver",
|
||||
],
|
||||
)
|
||||
|
||||
cc_library(
|
||||
name = "inference_calculator_cpu",
|
||||
srcs = [
|
||||
|
@ -232,22 +276,64 @@ cc_library(
|
|||
visibility = ["//visibility:public"],
|
||||
deps = [
|
||||
":inference_calculator_interface",
|
||||
":inference_calculator_utils",
|
||||
":inference_interpreter_delegate_runner",
|
||||
":inference_runner",
|
||||
"@com_google_absl//absl/memory",
|
||||
"@com_google_absl//absl/status",
|
||||
"@com_google_absl//absl/status:statusor",
|
||||
"@org_tensorflow//tensorflow/lite/delegates/xnnpack:xnnpack_delegate",
|
||||
"@org_tensorflow//tensorflow/lite:framework_stable",
|
||||
"@org_tensorflow//tensorflow/lite/c:c_api_types",
|
||||
] + select({
|
||||
"//conditions:default": [
|
||||
"//mediapipe/util:cpu_util",
|
||||
],
|
||||
}) + select({
|
||||
"//conditions:default": [],
|
||||
"//mediapipe:android": ["@org_tensorflow//tensorflow/lite/delegates/nnapi:nnapi_delegate"],
|
||||
}),
|
||||
alwayslink = 1,
|
||||
)
|
||||
|
||||
cc_library(
|
||||
name = "inference_calculator_utils",
|
||||
srcs = ["inference_calculator_utils.cc"],
|
||||
hdrs = ["inference_calculator_utils.h"],
|
||||
deps = [
|
||||
":inference_calculator_cc_proto",
|
||||
"//mediapipe/framework:port",
|
||||
] + select({
|
||||
"//conditions:default": [
|
||||
"//mediapipe/util:cpu_util",
|
||||
],
|
||||
}),
|
||||
alwayslink = 1,
|
||||
)
|
||||
|
||||
cc_library(
|
||||
name = "inference_calculator_xnnpack",
|
||||
srcs = [
|
||||
"inference_calculator_xnnpack.cc",
|
||||
],
|
||||
copts = select({
|
||||
# TODO: fix tensor.h not to require this, if possible
|
||||
"//mediapipe:apple": [
|
||||
"-x objective-c++",
|
||||
"-fobjc-arc", # enable reference-counting
|
||||
],
|
||||
"//conditions:default": [],
|
||||
}),
|
||||
visibility = ["//visibility:public"],
|
||||
deps = [
|
||||
":inference_calculator_interface",
|
||||
":inference_calculator_utils",
|
||||
":inference_interpreter_delegate_runner",
|
||||
":inference_runner",
|
||||
"@com_google_absl//absl/status",
|
||||
"@com_google_absl//absl/status:statusor",
|
||||
"@org_tensorflow//tensorflow/lite:framework_stable",
|
||||
"@org_tensorflow//tensorflow/lite/delegates/xnnpack:xnnpack_delegate",
|
||||
],
|
||||
alwayslink = 1,
|
||||
)
|
||||
|
||||
cc_library(
|
||||
name = "inference_calculator_gl_if_compute_shader_available",
|
||||
visibility = ["//visibility:public"],
|
||||
|
|
|
@ -59,6 +59,7 @@ class InferenceCalculatorSelectorImpl
|
|||
}
|
||||
}
|
||||
impls.emplace_back("Cpu");
|
||||
impls.emplace_back("Xnnpack");
|
||||
for (const auto& suffix : impls) {
|
||||
const auto impl = absl::StrCat("InferenceCalculator", suffix);
|
||||
if (!mediapipe::CalculatorBaseRegistry::IsRegistered(impl)) continue;
|
||||
|
|
|
@ -141,6 +141,10 @@ struct InferenceCalculatorCpu : public InferenceCalculator {
|
|||
static constexpr char kCalculatorName[] = "InferenceCalculatorCpu";
|
||||
};
|
||||
|
||||
struct InferenceCalculatorXnnpack : public InferenceCalculator {
|
||||
static constexpr char kCalculatorName[] = "InferenceCalculatorXnnpack";
|
||||
};
|
||||
|
||||
} // namespace api2
|
||||
} // namespace mediapipe
|
||||
|
||||
|
|
|
@ -18,78 +18,21 @@
|
|||
#include <string>
|
||||
#include <vector>
|
||||
|
||||
#include "absl/memory/memory.h"
|
||||
#include "absl/status/status.h"
|
||||
#include "absl/status/statusor.h"
|
||||
#include "mediapipe/calculators/tensor/inference_calculator.h"
|
||||
#include "mediapipe/calculators/tensor/inference_calculator_utils.h"
|
||||
#include "mediapipe/calculators/tensor/inference_interpreter_delegate_runner.h"
|
||||
#include "mediapipe/calculators/tensor/inference_runner.h"
|
||||
#include "tensorflow/lite/interpreter.h"
|
||||
#include "tensorflow/lite/interpreter_builder.h"
|
||||
#if defined(MEDIAPIPE_ANDROID)
|
||||
#include "tensorflow/lite/delegates/nnapi/nnapi_delegate.h"
|
||||
#endif // ANDROID
|
||||
|
||||
#if !defined(__EMSCRIPTEN__) || defined(__EMSCRIPTEN_PTHREADS__)
|
||||
#include "mediapipe/util/cpu_util.h"
|
||||
#endif // !__EMSCRIPTEN__ || __EMSCRIPTEN_PTHREADS__
|
||||
|
||||
#include "tensorflow/lite/c/c_api_types.h"
|
||||
#include "tensorflow/lite/delegates/xnnpack/xnnpack_delegate.h"
|
||||
|
||||
namespace mediapipe {
|
||||
namespace api2 {
|
||||
|
||||
namespace {
|
||||
|
||||
int GetXnnpackDefaultNumThreads() {
|
||||
#if defined(MEDIAPIPE_ANDROID) || defined(MEDIAPIPE_IOS) || \
|
||||
defined(__EMSCRIPTEN_PTHREADS__)
|
||||
constexpr int kMinNumThreadsByDefault = 1;
|
||||
constexpr int kMaxNumThreadsByDefault = 4;
|
||||
return std::clamp(NumCPUCores() / 2, kMinNumThreadsByDefault,
|
||||
kMaxNumThreadsByDefault);
|
||||
#else
|
||||
return 1;
|
||||
#endif // MEDIAPIPE_ANDROID || MEDIAPIPE_IOS || __EMSCRIPTEN_PTHREADS__
|
||||
}
|
||||
|
||||
// Returns number of threads to configure XNNPACK delegate with.
|
||||
// Returns user provided value if specified. Otherwise, tries to choose optimal
|
||||
// number of threads depending on the device.
|
||||
int GetXnnpackNumThreads(
|
||||
const bool opts_has_delegate,
|
||||
const mediapipe::InferenceCalculatorOptions::Delegate& opts_delegate) {
|
||||
static constexpr int kDefaultNumThreads = -1;
|
||||
if (opts_has_delegate && opts_delegate.has_xnnpack() &&
|
||||
opts_delegate.xnnpack().num_threads() != kDefaultNumThreads) {
|
||||
return opts_delegate.xnnpack().num_threads();
|
||||
}
|
||||
return GetXnnpackDefaultNumThreads();
|
||||
}
|
||||
|
||||
template <typename T>
|
||||
void CopyTensorBufferToInterpreter(const Tensor& input_tensor,
|
||||
tflite::Interpreter* interpreter,
|
||||
int input_tensor_index) {
|
||||
auto input_tensor_view = input_tensor.GetCpuReadView();
|
||||
auto input_tensor_buffer = input_tensor_view.buffer<T>();
|
||||
T* local_tensor_buffer =
|
||||
interpreter->typed_input_tensor<T>(input_tensor_index);
|
||||
std::memcpy(local_tensor_buffer, input_tensor_buffer, input_tensor.bytes());
|
||||
}
|
||||
|
||||
template <typename T>
|
||||
void CopyTensorBufferFromInterpreter(tflite::Interpreter* interpreter,
|
||||
int output_tensor_index,
|
||||
Tensor* output_tensor) {
|
||||
auto output_tensor_view = output_tensor->GetCpuWriteView();
|
||||
auto output_tensor_buffer = output_tensor_view.buffer<T>();
|
||||
T* local_tensor_buffer =
|
||||
interpreter->typed_output_tensor<T>(output_tensor_index);
|
||||
std::memcpy(output_tensor_buffer, local_tensor_buffer,
|
||||
output_tensor->bytes());
|
||||
}
|
||||
|
||||
} // namespace
|
||||
|
||||
class InferenceCalculatorCpuImpl
|
||||
: public NodeImpl<InferenceCalculatorCpu, InferenceCalculatorCpuImpl> {
|
||||
public:
|
||||
|
@ -100,16 +43,11 @@ class InferenceCalculatorCpuImpl
|
|||
absl::Status Close(CalculatorContext* cc) override;
|
||||
|
||||
private:
|
||||
absl::Status InitInterpreter(CalculatorContext* cc);
|
||||
absl::Status LoadDelegate(CalculatorContext* cc,
|
||||
tflite::InterpreterBuilder* interpreter_builder);
|
||||
absl::Status AllocateTensors();
|
||||
absl::StatusOr<std::unique_ptr<InferenceRunner>> CreateInferenceRunner(
|
||||
CalculatorContext* cc);
|
||||
absl::StatusOr<TfLiteDelegatePtr> MaybeCreateDelegate(CalculatorContext* cc);
|
||||
|
||||
// TfLite requires us to keep the model alive as long as the interpreter is.
|
||||
Packet<TfLiteModelPtr> model_packet_;
|
||||
std::unique_ptr<tflite::Interpreter> interpreter_;
|
||||
TfLiteDelegatePtr delegate_;
|
||||
TfLiteType input_tensor_type_ = TfLiteType::kTfLiteNoType;
|
||||
std::unique_ptr<InferenceRunner> inference_runner_;
|
||||
};
|
||||
|
||||
absl::Status InferenceCalculatorCpuImpl::UpdateContract(
|
||||
|
@ -122,7 +60,8 @@ absl::Status InferenceCalculatorCpuImpl::UpdateContract(
|
|||
}
|
||||
|
||||
absl::Status InferenceCalculatorCpuImpl::Open(CalculatorContext* cc) {
|
||||
return InitInterpreter(cc);
|
||||
ASSIGN_OR_RETURN(inference_runner_, CreateInferenceRunner(cc));
|
||||
return absl::OkStatus();
|
||||
}
|
||||
|
||||
absl::Status InferenceCalculatorCpuImpl::Process(CalculatorContext* cc) {
|
||||
|
@ -131,123 +70,32 @@ absl::Status InferenceCalculatorCpuImpl::Process(CalculatorContext* cc) {
|
|||
}
|
||||
const auto& input_tensors = *kInTensors(cc);
|
||||
RET_CHECK(!input_tensors.empty());
|
||||
auto output_tensors = absl::make_unique<std::vector<Tensor>>();
|
||||
|
||||
if (input_tensor_type_ == kTfLiteNoType) {
|
||||
input_tensor_type_ = interpreter_->tensor(interpreter_->inputs()[0])->type;
|
||||
}
|
||||
|
||||
// Read CPU input into tensors.
|
||||
for (int i = 0; i < input_tensors.size(); ++i) {
|
||||
switch (input_tensor_type_) {
|
||||
case TfLiteType::kTfLiteFloat16:
|
||||
case TfLiteType::kTfLiteFloat32: {
|
||||
CopyTensorBufferToInterpreter<float>(input_tensors[i],
|
||||
interpreter_.get(), i);
|
||||
break;
|
||||
}
|
||||
case TfLiteType::kTfLiteUInt8: {
|
||||
CopyTensorBufferToInterpreter<uint8>(input_tensors[i],
|
||||
interpreter_.get(), i);
|
||||
break;
|
||||
}
|
||||
case TfLiteType::kTfLiteInt8: {
|
||||
CopyTensorBufferToInterpreter<int8>(input_tensors[i],
|
||||
interpreter_.get(), i);
|
||||
break;
|
||||
}
|
||||
case TfLiteType::kTfLiteInt32: {
|
||||
CopyTensorBufferToInterpreter<int32_t>(input_tensors[i],
|
||||
interpreter_.get(), i);
|
||||
break;
|
||||
}
|
||||
default:
|
||||
return absl::InvalidArgumentError(
|
||||
absl::StrCat("Unsupported input tensor type:", input_tensor_type_));
|
||||
}
|
||||
}
|
||||
|
||||
// Run inference.
|
||||
RET_CHECK_EQ(interpreter_->Invoke(), kTfLiteOk);
|
||||
|
||||
// Output result tensors (CPU).
|
||||
const auto& tensor_indexes = interpreter_->outputs();
|
||||
output_tensors->reserve(tensor_indexes.size());
|
||||
for (int i = 0; i < tensor_indexes.size(); ++i) {
|
||||
TfLiteTensor* tensor = interpreter_->tensor(tensor_indexes[i]);
|
||||
Tensor::Shape shape{std::vector<int>{
|
||||
tensor->dims->data, tensor->dims->data + tensor->dims->size}};
|
||||
switch (tensor->type) {
|
||||
case TfLiteType::kTfLiteFloat16:
|
||||
case TfLiteType::kTfLiteFloat32:
|
||||
output_tensors->emplace_back(Tensor::ElementType::kFloat32, shape);
|
||||
CopyTensorBufferFromInterpreter<float>(interpreter_.get(), i,
|
||||
&output_tensors->back());
|
||||
break;
|
||||
case TfLiteType::kTfLiteUInt8:
|
||||
output_tensors->emplace_back(
|
||||
Tensor::ElementType::kUInt8, shape,
|
||||
Tensor::QuantizationParameters{tensor->params.scale,
|
||||
tensor->params.zero_point});
|
||||
CopyTensorBufferFromInterpreter<uint8>(interpreter_.get(), i,
|
||||
&output_tensors->back());
|
||||
break;
|
||||
case TfLiteType::kTfLiteInt8:
|
||||
output_tensors->emplace_back(
|
||||
Tensor::ElementType::kInt8, shape,
|
||||
Tensor::QuantizationParameters{tensor->params.scale,
|
||||
tensor->params.zero_point});
|
||||
CopyTensorBufferFromInterpreter<int8>(interpreter_.get(), i,
|
||||
&output_tensors->back());
|
||||
break;
|
||||
case TfLiteType::kTfLiteInt32:
|
||||
output_tensors->emplace_back(Tensor::ElementType::kInt32, shape);
|
||||
CopyTensorBufferFromInterpreter<int32_t>(interpreter_.get(), i,
|
||||
&output_tensors->back());
|
||||
break;
|
||||
default:
|
||||
return absl::InvalidArgumentError(
|
||||
absl::StrCat("Unsupported output tensor type:",
|
||||
TfLiteTypeGetName(tensor->type)));
|
||||
}
|
||||
}
|
||||
ASSIGN_OR_RETURN(std::vector<Tensor> output_tensors,
|
||||
inference_runner_->Run(input_tensors));
|
||||
kOutTensors(cc).Send(std::move(output_tensors));
|
||||
return absl::OkStatus();
|
||||
}
|
||||
|
||||
absl::Status InferenceCalculatorCpuImpl::Close(CalculatorContext* cc) {
|
||||
interpreter_ = nullptr;
|
||||
delegate_ = nullptr;
|
||||
inference_runner_ = nullptr;
|
||||
return absl::OkStatus();
|
||||
}
|
||||
|
||||
absl::Status InferenceCalculatorCpuImpl::InitInterpreter(
|
||||
CalculatorContext* cc) {
|
||||
ASSIGN_OR_RETURN(model_packet_, GetModelAsPacket(cc));
|
||||
const auto& model = *model_packet_.Get();
|
||||
absl::StatusOr<std::unique_ptr<InferenceRunner>>
|
||||
InferenceCalculatorCpuImpl::CreateInferenceRunner(CalculatorContext* cc) {
|
||||
ASSIGN_OR_RETURN(auto model_packet, GetModelAsPacket(cc));
|
||||
ASSIGN_OR_RETURN(auto op_resolver_packet, GetOpResolverAsPacket(cc));
|
||||
const auto& op_resolver = op_resolver_packet.Get();
|
||||
tflite::InterpreterBuilder interpreter_builder(model, op_resolver);
|
||||
MP_RETURN_IF_ERROR(LoadDelegate(cc, &interpreter_builder));
|
||||
#if defined(__EMSCRIPTEN__)
|
||||
interpreter_builder.SetNumThreads(1);
|
||||
#else
|
||||
interpreter_builder.SetNumThreads(
|
||||
cc->Options<mediapipe::InferenceCalculatorOptions>().cpu_num_thread());
|
||||
#endif // __EMSCRIPTEN__
|
||||
|
||||
RET_CHECK_EQ(interpreter_builder(&interpreter_), kTfLiteOk);
|
||||
RET_CHECK(interpreter_);
|
||||
return AllocateTensors();
|
||||
const int interpreter_num_threads =
|
||||
cc->Options<mediapipe::InferenceCalculatorOptions>().cpu_num_thread();
|
||||
ASSIGN_OR_RETURN(TfLiteDelegatePtr delegate, MaybeCreateDelegate(cc));
|
||||
return CreateInferenceInterpreterDelegateRunner(
|
||||
std::move(model_packet), std::move(op_resolver_packet),
|
||||
std::move(delegate), interpreter_num_threads);
|
||||
}
|
||||
|
||||
absl::Status InferenceCalculatorCpuImpl::AllocateTensors() {
|
||||
RET_CHECK_EQ(interpreter_->AllocateTensors(), kTfLiteOk);
|
||||
return absl::OkStatus();
|
||||
}
|
||||
|
||||
absl::Status InferenceCalculatorCpuImpl::LoadDelegate(
|
||||
CalculatorContext* cc, tflite::InterpreterBuilder* interpreter_builder) {
|
||||
absl::StatusOr<TfLiteDelegatePtr>
|
||||
InferenceCalculatorCpuImpl::MaybeCreateDelegate(CalculatorContext* cc) {
|
||||
const auto& calculator_opts =
|
||||
cc->Options<mediapipe::InferenceCalculatorOptions>();
|
||||
auto opts_delegate = calculator_opts.delegate();
|
||||
|
@ -268,7 +116,7 @@ absl::Status InferenceCalculatorCpuImpl::LoadDelegate(
|
|||
calculator_opts.has_delegate() || !kDelegate(cc).IsEmpty();
|
||||
if (opts_has_delegate && opts_delegate.has_tflite()) {
|
||||
// Default tflite inference requeqsted - no need to modify graph.
|
||||
return absl::OkStatus();
|
||||
return nullptr;
|
||||
}
|
||||
|
||||
#if defined(MEDIAPIPE_ANDROID)
|
||||
|
@ -288,10 +136,8 @@ absl::Status InferenceCalculatorCpuImpl::LoadDelegate(
|
|||
options.accelerator_name = nnapi.has_accelerator_name()
|
||||
? nnapi.accelerator_name().c_str()
|
||||
: nullptr;
|
||||
delegate_ = TfLiteDelegatePtr(new tflite::StatefulNnApiDelegate(options),
|
||||
[](TfLiteDelegate*) {});
|
||||
interpreter_builder->AddDelegate(delegate_.get());
|
||||
return absl::OkStatus();
|
||||
return TfLiteDelegatePtr(new tflite::StatefulNnApiDelegate(options),
|
||||
[](TfLiteDelegate*) {});
|
||||
}
|
||||
#endif // MEDIAPIPE_ANDROID
|
||||
|
||||
|
@ -305,12 +151,11 @@ absl::Status InferenceCalculatorCpuImpl::LoadDelegate(
|
|||
auto xnnpack_opts = TfLiteXNNPackDelegateOptionsDefault();
|
||||
xnnpack_opts.num_threads =
|
||||
GetXnnpackNumThreads(opts_has_delegate, opts_delegate);
|
||||
delegate_ = TfLiteDelegatePtr(TfLiteXNNPackDelegateCreate(&xnnpack_opts),
|
||||
&TfLiteXNNPackDelegateDelete);
|
||||
interpreter_builder->AddDelegate(delegate_.get());
|
||||
return TfLiteDelegatePtr(TfLiteXNNPackDelegateCreate(&xnnpack_opts),
|
||||
&TfLiteXNNPackDelegateDelete);
|
||||
}
|
||||
|
||||
return absl::OkStatus();
|
||||
return nullptr;
|
||||
}
|
||||
|
||||
} // namespace api2
|
||||
|
|
53
mediapipe/calculators/tensor/inference_calculator_utils.cc
Normal file
53
mediapipe/calculators/tensor/inference_calculator_utils.cc
Normal file
|
@ -0,0 +1,53 @@
|
|||
// Copyright 2022 The MediaPipe Authors.
|
||||
//
|
||||
// Licensed under the Apache License, Version 2.0 (the "License");
|
||||
// you may not use this file except in compliance with the License.
|
||||
// You may obtain a copy of the License at
|
||||
//
|
||||
// http://www.apache.org/licenses/LICENSE-2.0
|
||||
//
|
||||
// Unless required by applicable law or agreed to in writing, software
|
||||
// distributed under the License is distributed on an "AS IS" BASIS,
|
||||
// WITHOUT WARRANTIES OR CONDITIONS OF ANY KIND, either express or implied.
|
||||
// See the License for the specific language governing permissions and
|
||||
// limitations under the License.
|
||||
|
||||
#include "mediapipe/calculators/tensor/inference_calculator_utils.h"
|
||||
|
||||
#include "mediapipe/calculators/tensor/inference_calculator.pb.h"
|
||||
#include "mediapipe/framework/port.h" // NOLINT: provides MEDIAPIPE_ANDROID/IOS
|
||||
|
||||
#if !defined(__EMSCRIPTEN__) || defined(__EMSCRIPTEN_PTHREADS__)
|
||||
#include "mediapipe/util/cpu_util.h"
|
||||
#endif // !__EMSCRIPTEN__ || __EMSCRIPTEN_PTHREADS__
|
||||
|
||||
namespace mediapipe {
|
||||
|
||||
namespace {
|
||||
|
||||
int GetXnnpackDefaultNumThreads() {
|
||||
#if defined(MEDIAPIPE_ANDROID) || defined(MEDIAPIPE_IOS) || \
|
||||
defined(__EMSCRIPTEN_PTHREADS__)
|
||||
constexpr int kMinNumThreadsByDefault = 1;
|
||||
constexpr int kMaxNumThreadsByDefault = 4;
|
||||
return std::clamp(NumCPUCores() / 2, kMinNumThreadsByDefault,
|
||||
kMaxNumThreadsByDefault);
|
||||
#else
|
||||
return 1;
|
||||
#endif // MEDIAPIPE_ANDROID || MEDIAPIPE_IOS || __EMSCRIPTEN_PTHREADS__
|
||||
}
|
||||
|
||||
} // namespace
|
||||
|
||||
int GetXnnpackNumThreads(
|
||||
const bool opts_has_delegate,
|
||||
const mediapipe::InferenceCalculatorOptions::Delegate& opts_delegate) {
|
||||
static constexpr int kDefaultNumThreads = -1;
|
||||
if (opts_has_delegate && opts_delegate.has_xnnpack() &&
|
||||
opts_delegate.xnnpack().num_threads() != kDefaultNumThreads) {
|
||||
return opts_delegate.xnnpack().num_threads();
|
||||
}
|
||||
return GetXnnpackDefaultNumThreads();
|
||||
}
|
||||
|
||||
} // namespace mediapipe
|
31
mediapipe/calculators/tensor/inference_calculator_utils.h
Normal file
31
mediapipe/calculators/tensor/inference_calculator_utils.h
Normal file
|
@ -0,0 +1,31 @@
|
|||
// Copyright 2022 The MediaPipe Authors.
|
||||
//
|
||||
// Licensed under the Apache License, Version 2.0 (the "License");
|
||||
// you may not use this file except in compliance with the License.
|
||||
// You may obtain a copy of the License at
|
||||
//
|
||||
// http://www.apache.org/licenses/LICENSE-2.0
|
||||
//
|
||||
// Unless required by applicable law or agreed to in writing, software
|
||||
// distributed under the License is distributed on an "AS IS" BASIS,
|
||||
// WITHOUT WARRANTIES OR CONDITIONS OF ANY KIND, either express or implied.
|
||||
// See the License for the specific language governing permissions and
|
||||
// limitations under the License.
|
||||
|
||||
#ifndef MEDIAPIPE_CALCULATORS_TENSOR_INFERENCE_CALCULATOR_UTILS_H_
|
||||
#define MEDIAPIPE_CALCULATORS_TENSOR_INFERENCE_CALCULATOR_UTILS_H_
|
||||
|
||||
#include "mediapipe/calculators/tensor/inference_calculator.pb.h"
|
||||
|
||||
namespace mediapipe {
|
||||
|
||||
// Returns number of threads to configure XNNPACK delegate with.
|
||||
// Returns user provided value if specified. Otherwise, tries to choose optimal
|
||||
// number of threads depending on the device.
|
||||
int GetXnnpackNumThreads(
|
||||
const bool opts_has_delegate,
|
||||
const mediapipe::InferenceCalculatorOptions::Delegate& opts_delegate);
|
||||
|
||||
} // namespace mediapipe
|
||||
|
||||
#endif // MEDIAPIPE_CALCULATORS_TENSOR_INFERENCE_CALCULATOR_UTILS_H_
|
122
mediapipe/calculators/tensor/inference_calculator_xnnpack.cc
Normal file
122
mediapipe/calculators/tensor/inference_calculator_xnnpack.cc
Normal file
|
@ -0,0 +1,122 @@
|
|||
// Copyright 2022 The MediaPipe Authors.
|
||||
//
|
||||
// Licensed under the Apache License, Version 2.0 (the "License");
|
||||
// you may not use this file except in compliance with the License.
|
||||
// You may obtain a copy of the License at
|
||||
//
|
||||
// http://www.apache.org/licenses/LICENSE-2.0
|
||||
//
|
||||
// Unless required by applicable law or agreed to in writing, software
|
||||
// distributed under the License is distributed on an "AS IS" BASIS,
|
||||
// WITHOUT WARRANTIES OR CONDITIONS OF ANY KIND, either express or implied.
|
||||
// See the License for the specific language governing permissions and
|
||||
// limitations under the License.
|
||||
|
||||
#include <cstdint>
|
||||
#include <cstring>
|
||||
#include <memory>
|
||||
#include <string>
|
||||
#include <vector>
|
||||
|
||||
#include "absl/status/status.h"
|
||||
#include "absl/status/statusor.h"
|
||||
#include "mediapipe/calculators/tensor/inference_calculator.h"
|
||||
#include "mediapipe/calculators/tensor/inference_calculator_utils.h"
|
||||
#include "mediapipe/calculators/tensor/inference_interpreter_delegate_runner.h"
|
||||
#include "mediapipe/calculators/tensor/inference_runner.h"
|
||||
#include "tensorflow/lite/delegates/xnnpack/xnnpack_delegate.h"
|
||||
#include "tensorflow/lite/interpreter.h"
|
||||
|
||||
namespace mediapipe {
|
||||
namespace api2 {
|
||||
|
||||
class InferenceCalculatorXnnpackImpl
|
||||
: public NodeImpl<InferenceCalculatorXnnpack,
|
||||
InferenceCalculatorXnnpackImpl> {
|
||||
public:
|
||||
static absl::Status UpdateContract(CalculatorContract* cc);
|
||||
|
||||
absl::Status Open(CalculatorContext* cc) override;
|
||||
absl::Status Process(CalculatorContext* cc) override;
|
||||
absl::Status Close(CalculatorContext* cc) override;
|
||||
|
||||
private:
|
||||
absl::StatusOr<std::unique_ptr<InferenceRunner>> CreateInferenceRunner(
|
||||
CalculatorContext* cc);
|
||||
absl::StatusOr<TfLiteDelegatePtr> CreateDelegate(CalculatorContext* cc);
|
||||
|
||||
std::unique_ptr<InferenceRunner> inference_runner_;
|
||||
};
|
||||
|
||||
absl::Status InferenceCalculatorXnnpackImpl::UpdateContract(
|
||||
CalculatorContract* cc) {
|
||||
const auto& options = cc->Options<mediapipe::InferenceCalculatorOptions>();
|
||||
RET_CHECK(!options.model_path().empty() ^ kSideInModel(cc).IsConnected())
|
||||
<< "Either model as side packet or model path in options is required.";
|
||||
|
||||
return absl::OkStatus();
|
||||
}
|
||||
|
||||
absl::Status InferenceCalculatorXnnpackImpl::Open(CalculatorContext* cc) {
|
||||
ASSIGN_OR_RETURN(inference_runner_, CreateInferenceRunner(cc));
|
||||
return absl::OkStatus();
|
||||
}
|
||||
|
||||
absl::Status InferenceCalculatorXnnpackImpl::Process(CalculatorContext* cc) {
|
||||
if (kInTensors(cc).IsEmpty()) {
|
||||
return absl::OkStatus();
|
||||
}
|
||||
const auto& input_tensors = *kInTensors(cc);
|
||||
RET_CHECK(!input_tensors.empty());
|
||||
|
||||
ASSIGN_OR_RETURN(std::vector<Tensor> output_tensors,
|
||||
inference_runner_->Run(input_tensors));
|
||||
kOutTensors(cc).Send(std::move(output_tensors));
|
||||
return absl::OkStatus();
|
||||
}
|
||||
|
||||
absl::Status InferenceCalculatorXnnpackImpl::Close(CalculatorContext* cc) {
|
||||
inference_runner_ = nullptr;
|
||||
return absl::OkStatus();
|
||||
}
|
||||
|
||||
absl::StatusOr<std::unique_ptr<InferenceRunner>>
|
||||
InferenceCalculatorXnnpackImpl::CreateInferenceRunner(CalculatorContext* cc) {
|
||||
ASSIGN_OR_RETURN(auto model_packet, GetModelAsPacket(cc));
|
||||
ASSIGN_OR_RETURN(auto op_resolver_packet, GetOpResolverAsPacket(cc));
|
||||
const int interpreter_num_threads =
|
||||
cc->Options<mediapipe::InferenceCalculatorOptions>().cpu_num_thread();
|
||||
ASSIGN_OR_RETURN(TfLiteDelegatePtr delegate, CreateDelegate(cc));
|
||||
return CreateInferenceInterpreterDelegateRunner(
|
||||
std::move(model_packet), std::move(op_resolver_packet),
|
||||
std::move(delegate), interpreter_num_threads);
|
||||
}
|
||||
|
||||
absl::StatusOr<TfLiteDelegatePtr>
|
||||
InferenceCalculatorXnnpackImpl::CreateDelegate(CalculatorContext* cc) {
|
||||
const auto& calculator_opts =
|
||||
cc->Options<mediapipe::InferenceCalculatorOptions>();
|
||||
auto opts_delegate = calculator_opts.delegate();
|
||||
if (!kDelegate(cc).IsEmpty()) {
|
||||
const mediapipe::InferenceCalculatorOptions::Delegate&
|
||||
input_side_packet_delegate = kDelegate(cc).Get();
|
||||
RET_CHECK(
|
||||
input_side_packet_delegate.has_xnnpack() ||
|
||||
input_side_packet_delegate.delegate_case() ==
|
||||
mediapipe::InferenceCalculatorOptions::Delegate::DELEGATE_NOT_SET)
|
||||
<< "inference_calculator_cpu only supports delegate input side packet "
|
||||
<< "for TFLite, XNNPack";
|
||||
opts_delegate.MergeFrom(input_side_packet_delegate);
|
||||
}
|
||||
const bool opts_has_delegate =
|
||||
calculator_opts.has_delegate() || !kDelegate(cc).IsEmpty();
|
||||
|
||||
auto xnnpack_opts = TfLiteXNNPackDelegateOptionsDefault();
|
||||
xnnpack_opts.num_threads =
|
||||
GetXnnpackNumThreads(opts_has_delegate, opts_delegate);
|
||||
return TfLiteDelegatePtr(TfLiteXNNPackDelegateCreate(&xnnpack_opts),
|
||||
&TfLiteXNNPackDelegateDelete);
|
||||
}
|
||||
|
||||
} // namespace api2
|
||||
} // namespace mediapipe
|
|
@ -0,0 +1,181 @@
|
|||
// Copyright 2022 The MediaPipe Authors.
|
||||
//
|
||||
// Licensed under the Apache License, Version 2.0 (the "License");
|
||||
// you may not use this file except in compliance with the License.
|
||||
// You may obtain a copy of the License at
|
||||
//
|
||||
// http://www.apache.org/licenses/LICENSE-2.0
|
||||
//
|
||||
// Unless required by applicable law or agreed to in writing, software
|
||||
// distributed under the License is distributed on an "AS IS" BASIS,
|
||||
// WITHOUT WARRANTIES OR CONDITIONS OF ANY KIND, either express or implied.
|
||||
// See the License for the specific language governing permissions and
|
||||
// limitations under the License.
|
||||
|
||||
#include "mediapipe/calculators/tensor/inference_interpreter_delegate_runner.h"
|
||||
|
||||
#include <memory>
|
||||
#include <vector>
|
||||
|
||||
#include "absl/status/status.h"
|
||||
#include "absl/status/statusor.h"
|
||||
#include "mediapipe/framework/formats/tensor.h"
|
||||
#include "mediapipe/framework/port/ret_check.h"
|
||||
#include "tensorflow/lite/interpreter.h"
|
||||
#include "tensorflow/lite/interpreter_builder.h"
|
||||
|
||||
namespace mediapipe {
|
||||
|
||||
namespace {
|
||||
|
||||
template <typename T>
|
||||
void CopyTensorBufferToInterpreter(const Tensor& input_tensor,
|
||||
tflite::Interpreter* interpreter,
|
||||
int input_tensor_index) {
|
||||
auto input_tensor_view = input_tensor.GetCpuReadView();
|
||||
auto input_tensor_buffer = input_tensor_view.buffer<T>();
|
||||
T* local_tensor_buffer =
|
||||
interpreter->typed_input_tensor<T>(input_tensor_index);
|
||||
std::memcpy(local_tensor_buffer, input_tensor_buffer, input_tensor.bytes());
|
||||
}
|
||||
|
||||
template <typename T>
|
||||
void CopyTensorBufferFromInterpreter(tflite::Interpreter* interpreter,
|
||||
int output_tensor_index,
|
||||
Tensor* output_tensor) {
|
||||
auto output_tensor_view = output_tensor->GetCpuWriteView();
|
||||
auto output_tensor_buffer = output_tensor_view.buffer<T>();
|
||||
T* local_tensor_buffer =
|
||||
interpreter->typed_output_tensor<T>(output_tensor_index);
|
||||
std::memcpy(output_tensor_buffer, local_tensor_buffer,
|
||||
output_tensor->bytes());
|
||||
}
|
||||
|
||||
} // namespace
|
||||
|
||||
class InferenceInterpreterDelegateRunner : public InferenceRunner {
|
||||
public:
|
||||
InferenceInterpreterDelegateRunner(
|
||||
api2::Packet<TfLiteModelPtr> model,
|
||||
std::unique_ptr<tflite::Interpreter> interpreter,
|
||||
TfLiteDelegatePtr delegate)
|
||||
: model_(std::move(model)),
|
||||
interpreter_(std::move(interpreter)),
|
||||
delegate_(std::move(delegate)) {}
|
||||
|
||||
absl::StatusOr<std::vector<Tensor>> Run(
|
||||
const std::vector<Tensor>& input_tensors) override;
|
||||
|
||||
private:
|
||||
api2::Packet<TfLiteModelPtr> model_;
|
||||
std::unique_ptr<tflite::Interpreter> interpreter_;
|
||||
TfLiteDelegatePtr delegate_;
|
||||
};
|
||||
|
||||
absl::StatusOr<std::vector<Tensor>> InferenceInterpreterDelegateRunner::Run(
|
||||
const std::vector<Tensor>& input_tensors) {
|
||||
// Read CPU input into tensors.
|
||||
RET_CHECK_EQ(interpreter_->inputs().size(), input_tensors.size());
|
||||
for (int i = 0; i < input_tensors.size(); ++i) {
|
||||
const TfLiteType input_tensor_type =
|
||||
interpreter_->tensor(interpreter_->inputs()[i])->type;
|
||||
switch (input_tensor_type) {
|
||||
case TfLiteType::kTfLiteFloat16:
|
||||
case TfLiteType::kTfLiteFloat32: {
|
||||
CopyTensorBufferToInterpreter<float>(input_tensors[i],
|
||||
interpreter_.get(), i);
|
||||
break;
|
||||
}
|
||||
case TfLiteType::kTfLiteUInt8: {
|
||||
CopyTensorBufferToInterpreter<uint8>(input_tensors[i],
|
||||
interpreter_.get(), i);
|
||||
break;
|
||||
}
|
||||
case TfLiteType::kTfLiteInt8: {
|
||||
CopyTensorBufferToInterpreter<int8>(input_tensors[i],
|
||||
interpreter_.get(), i);
|
||||
break;
|
||||
}
|
||||
case TfLiteType::kTfLiteInt32: {
|
||||
CopyTensorBufferToInterpreter<int32_t>(input_tensors[i],
|
||||
interpreter_.get(), i);
|
||||
break;
|
||||
}
|
||||
default:
|
||||
return absl::InvalidArgumentError(
|
||||
absl::StrCat("Unsupported input tensor type:", input_tensor_type));
|
||||
}
|
||||
}
|
||||
|
||||
// Run inference.
|
||||
RET_CHECK_EQ(interpreter_->Invoke(), kTfLiteOk);
|
||||
|
||||
// Output result tensors (CPU).
|
||||
const auto& tensor_indexes = interpreter_->outputs();
|
||||
std::vector<Tensor> output_tensors;
|
||||
output_tensors.reserve(tensor_indexes.size());
|
||||
for (int i = 0; i < tensor_indexes.size(); ++i) {
|
||||
TfLiteTensor* tensor = interpreter_->tensor(tensor_indexes[i]);
|
||||
Tensor::Shape shape{std::vector<int>{
|
||||
tensor->dims->data, tensor->dims->data + tensor->dims->size}};
|
||||
switch (tensor->type) {
|
||||
case TfLiteType::kTfLiteFloat16:
|
||||
case TfLiteType::kTfLiteFloat32:
|
||||
output_tensors.emplace_back(Tensor::ElementType::kFloat32, shape);
|
||||
CopyTensorBufferFromInterpreter<float>(interpreter_.get(), i,
|
||||
&output_tensors.back());
|
||||
break;
|
||||
case TfLiteType::kTfLiteUInt8:
|
||||
output_tensors.emplace_back(
|
||||
Tensor::ElementType::kUInt8, shape,
|
||||
Tensor::QuantizationParameters{tensor->params.scale,
|
||||
tensor->params.zero_point});
|
||||
CopyTensorBufferFromInterpreter<uint8>(interpreter_.get(), i,
|
||||
&output_tensors.back());
|
||||
break;
|
||||
case TfLiteType::kTfLiteInt8:
|
||||
output_tensors.emplace_back(
|
||||
Tensor::ElementType::kInt8, shape,
|
||||
Tensor::QuantizationParameters{tensor->params.scale,
|
||||
tensor->params.zero_point});
|
||||
CopyTensorBufferFromInterpreter<int8>(interpreter_.get(), i,
|
||||
&output_tensors.back());
|
||||
break;
|
||||
case TfLiteType::kTfLiteInt32:
|
||||
output_tensors.emplace_back(Tensor::ElementType::kInt32, shape);
|
||||
CopyTensorBufferFromInterpreter<int32_t>(interpreter_.get(), i,
|
||||
&output_tensors.back());
|
||||
break;
|
||||
default:
|
||||
return absl::InvalidArgumentError(
|
||||
absl::StrCat("Unsupported output tensor type:",
|
||||
TfLiteTypeGetName(tensor->type)));
|
||||
}
|
||||
}
|
||||
return output_tensors;
|
||||
}
|
||||
|
||||
absl::StatusOr<std::unique_ptr<InferenceRunner>>
|
||||
CreateInferenceInterpreterDelegateRunner(
|
||||
api2::Packet<TfLiteModelPtr> model,
|
||||
api2::Packet<tflite::OpResolver> op_resolver, TfLiteDelegatePtr delegate,
|
||||
int interpreter_num_threads) {
|
||||
tflite::InterpreterBuilder interpreter_builder(*model.Get(),
|
||||
op_resolver.Get());
|
||||
if (delegate) {
|
||||
interpreter_builder.AddDelegate(delegate.get());
|
||||
}
|
||||
#if defined(__EMSCRIPTEN__)
|
||||
interpreter_builder.SetNumThreads(1);
|
||||
#else
|
||||
interpreter_builder.SetNumThreads(interpreter_num_threads);
|
||||
#endif // __EMSCRIPTEN__
|
||||
std::unique_ptr<tflite::Interpreter> interpreter;
|
||||
RET_CHECK_EQ(interpreter_builder(&interpreter), kTfLiteOk);
|
||||
RET_CHECK(interpreter);
|
||||
RET_CHECK_EQ(interpreter->AllocateTensors(), kTfLiteOk);
|
||||
return std::make_unique<InferenceInterpreterDelegateRunner>(
|
||||
std::move(model), std::move(interpreter), std::move(delegate));
|
||||
}
|
||||
|
||||
} // namespace mediapipe
|
|
@ -0,0 +1,46 @@
|
|||
// Copyright 2022 The MediaPipe Authors.
|
||||
//
|
||||
// Licensed under the Apache License, Version 2.0 (the "License");
|
||||
// you may not use this file except in compliance with the License.
|
||||
// You may obtain a copy of the License at
|
||||
//
|
||||
// http://www.apache.org/licenses/LICENSE-2.0
|
||||
//
|
||||
// Unless required by applicable law or agreed to in writing, software
|
||||
// distributed under the License is distributed on an "AS IS" BASIS,
|
||||
// WITHOUT WARRANTIES OR CONDITIONS OF ANY KIND, either express or implied.
|
||||
// See the License for the specific language governing permissions and
|
||||
// limitations under the License.
|
||||
|
||||
#ifndef MEDIAPIPE_CALCULATORS_TENSOR_INFERENCE_INTERPRETER_DELEGATE_RUNNER_H_
|
||||
#define MEDIAPIPE_CALCULATORS_TENSOR_INFERENCE_INTERPRETER_DELEGATE_RUNNER_H_
|
||||
|
||||
#include <memory>
|
||||
#include <vector>
|
||||
|
||||
#include "absl/status/statusor.h"
|
||||
#include "mediapipe/calculators/tensor/inference_runner.h"
|
||||
#include "mediapipe/framework/api2/packet.h"
|
||||
#include "mediapipe/util/tflite/tflite_model_loader.h"
|
||||
#include "tensorflow/lite/core/api/op_resolver.h"
|
||||
#include "tensorflow/lite/interpreter.h"
|
||||
|
||||
namespace mediapipe {
|
||||
|
||||
using TfLiteDelegatePtr =
|
||||
std::unique_ptr<TfLiteDelegate, std::function<void(TfLiteDelegate*)>>;
|
||||
|
||||
// Creates inference runner which run inference using newly initialized
|
||||
// interpreter and provided `delegate`.
|
||||
//
|
||||
// `delegate` can be nullptr, in that case newly initialized interpreter will
|
||||
// use what is available by default.
|
||||
absl::StatusOr<std::unique_ptr<InferenceRunner>>
|
||||
CreateInferenceInterpreterDelegateRunner(
|
||||
api2::Packet<TfLiteModelPtr> model,
|
||||
api2::Packet<tflite::OpResolver> op_resolver, TfLiteDelegatePtr delegate,
|
||||
int interpreter_num_threads);
|
||||
|
||||
} // namespace mediapipe
|
||||
|
||||
#endif // MEDIAPIPE_CALCULATORS_TENSOR_INFERENCE_INTERPRETER_DELEGATE_RUNNER_H_
|
19
mediapipe/calculators/tensor/inference_runner.h
Normal file
19
mediapipe/calculators/tensor/inference_runner.h
Normal file
|
@ -0,0 +1,19 @@
|
|||
#ifndef MEDIAPIPE_CALCULATORS_TENSOR_INFERENCE_RUNNER_H_
|
||||
#define MEDIAPIPE_CALCULATORS_TENSOR_INFERENCE_RUNNER_H_
|
||||
|
||||
#include "absl/status/statusor.h"
|
||||
#include "mediapipe/framework/formats/tensor.h"
|
||||
|
||||
namespace mediapipe {
|
||||
|
||||
// Common interface to implement inference runners in MediaPipe.
|
||||
class InferenceRunner {
|
||||
public:
|
||||
virtual ~InferenceRunner() = default;
|
||||
virtual absl::StatusOr<std::vector<Tensor>> Run(
|
||||
const std::vector<Tensor>& inputs) = 0;
|
||||
};
|
||||
|
||||
} // namespace mediapipe
|
||||
|
||||
#endif // MEDIAPIPE_CALCULATORS_TENSOR_INFERENCE_RUNNER_H_
|
Binary file not shown.
Before Width: | Height: | Size: 1.2 KiB After Width: | Height: | Size: 2.6 KiB |
Binary file not shown.
After Width: | Height: | Size: 1.2 KiB |
|
@ -84,6 +84,7 @@
|
|||
{
|
||||
"idiom" : "ipad",
|
||||
"size" : "76x76",
|
||||
"filename" : "76_c_Ipad_2x.png",
|
||||
"scale" : "2x"
|
||||
},
|
||||
{
|
||||
|
|
|
@ -324,6 +324,63 @@ TEST(BuilderTest, GraphIndexes) {
|
|||
EXPECT_THAT(graph.GetConfig(), EqualsProto(expected));
|
||||
}
|
||||
|
||||
class AnyAndSameTypeCalculator : public NodeIntf {
|
||||
public:
|
||||
static constexpr Input<AnyType> kAnyTypeInput{"INPUT"};
|
||||
static constexpr Output<AnyType> kAnyTypeOutput{"ANY_OUTPUT"};
|
||||
static constexpr Output<SameType<kAnyTypeInput>> kSameTypeOutput{
|
||||
"SAME_OUTPUT"};
|
||||
|
||||
static constexpr Input<int> kIntInput{"INT_INPUT"};
|
||||
// `SameType` usage for this output is only for testing purposes.
|
||||
//
|
||||
// `SameType` is designed to work with inputs of `AnyType` and, normally, you
|
||||
// would not use `Output<SameType<kIntInput>>` in a real calculator. You
|
||||
// should write `Output<int>` instead, since the type is known.
|
||||
static constexpr Output<SameType<kIntInput>> kSameIntOutput{
|
||||
"SAME_INT_OUTPUT"};
|
||||
|
||||
MEDIAPIPE_NODE_INTERFACE(AnyTypeCalculator, kAnyTypeInput, kAnyTypeOutput,
|
||||
kSameTypeOutput);
|
||||
};
|
||||
|
||||
TEST(BuilderTest, AnyAndSameTypeHandledProperly) {
|
||||
builder::Graph graph;
|
||||
builder::Source<internal::Generic> any_input =
|
||||
graph[Input<AnyType>{"GRAPH_ANY_INPUT"}];
|
||||
builder::Source<int> int_input = graph[Input<int>{"GRAPH_INT_INPUT"}];
|
||||
|
||||
auto& node = graph.AddNode("AnyAndSameTypeCalculator");
|
||||
any_input >> node[AnyAndSameTypeCalculator::kAnyTypeInput];
|
||||
int_input >> node[AnyAndSameTypeCalculator::kIntInput];
|
||||
|
||||
builder::Source<internal::Generic> any_type_output =
|
||||
node[AnyAndSameTypeCalculator::kAnyTypeOutput];
|
||||
any_type_output.SetName("any_type_output");
|
||||
|
||||
builder::Source<internal::Generic> same_type_output =
|
||||
node[AnyAndSameTypeCalculator::kSameTypeOutput];
|
||||
same_type_output.SetName("same_type_output");
|
||||
builder::Source<internal::Generic> same_int_output =
|
||||
node[AnyAndSameTypeCalculator::kSameIntOutput];
|
||||
same_int_output.SetName("same_int_output");
|
||||
|
||||
CalculatorGraphConfig expected =
|
||||
mediapipe::ParseTextProtoOrDie<CalculatorGraphConfig>(R"pb(
|
||||
node {
|
||||
calculator: "AnyAndSameTypeCalculator"
|
||||
input_stream: "INPUT:__stream_0"
|
||||
input_stream: "INT_INPUT:__stream_1"
|
||||
output_stream: "ANY_OUTPUT:any_type_output"
|
||||
output_stream: "SAME_INT_OUTPUT:same_int_output"
|
||||
output_stream: "SAME_OUTPUT:same_type_output"
|
||||
}
|
||||
input_stream: "GRAPH_ANY_INPUT:__stream_0"
|
||||
input_stream: "GRAPH_INT_INPUT:__stream_1"
|
||||
)pb");
|
||||
EXPECT_THAT(graph.GetConfig(), EqualsProto(expected));
|
||||
}
|
||||
|
||||
} // namespace test
|
||||
} // namespace api2
|
||||
} // namespace mediapipe
|
||||
|
|
|
@ -27,6 +27,12 @@ using HolderBase = mediapipe::packet_internal::HolderBase;
|
|||
template <typename T>
|
||||
class Packet;
|
||||
|
||||
struct DynamicType {};
|
||||
|
||||
struct AnyType : public DynamicType {
|
||||
AnyType() = delete;
|
||||
};
|
||||
|
||||
// Type-erased packet.
|
||||
class PacketBase {
|
||||
public:
|
||||
|
@ -148,9 +154,8 @@ inline void CheckCompatibleType(const HolderBase& holder,
|
|||
<< " was requested.";
|
||||
}
|
||||
|
||||
struct Generic {
|
||||
Generic() = delete;
|
||||
};
|
||||
// TODO: remove usage of internal::Generic and simply use AnyType.
|
||||
using Generic = ::mediapipe::api2::AnyType;
|
||||
|
||||
template <class V, class U>
|
||||
struct IsCompatibleType : std::false_type {};
|
||||
|
|
|
@ -77,10 +77,6 @@ struct NoneType {
|
|||
NoneType() = delete;
|
||||
};
|
||||
|
||||
struct DynamicType {};
|
||||
|
||||
struct AnyType : public DynamicType {};
|
||||
|
||||
template <auto& P>
|
||||
class SameType : public DynamicType {
|
||||
public:
|
||||
|
|
|
@ -103,6 +103,18 @@ public class ExternalTextureConverter implements TextureFrameProducer {
|
|||
}
|
||||
}
|
||||
|
||||
/**
|
||||
* Re-renders the current frame. Notifies all consumers as if it were a new frame. This should not
|
||||
* typically be used but can be useful for cases where the consumer has lost ownership of the most
|
||||
* recent frame and needs to get it again. This does nothing if no frame has yet been received.
|
||||
*/
|
||||
public void rerenderCurrentFrame() {
|
||||
SurfaceTexture surfaceTexture = getSurfaceTexture();
|
||||
if (thread != null && surfaceTexture != null && thread.getHasReceivedFirstFrame()) {
|
||||
thread.onFrameAvailable(surfaceTexture);
|
||||
}
|
||||
}
|
||||
|
||||
/**
|
||||
* Sets the new buffer pool size. This is safe to set at any time.
|
||||
*
|
||||
|
@ -278,6 +290,7 @@ public class ExternalTextureConverter implements TextureFrameProducer {
|
|||
private volatile SurfaceTexture internalSurfaceTexture = null;
|
||||
private int[] textures = null;
|
||||
private final List<TextureFrameConsumer> consumers;
|
||||
private volatile boolean hasReceivedFirstFrame = false;
|
||||
|
||||
private final Queue<PoolTextureFrame> framesAvailable = new ArrayDeque<>();
|
||||
private int framesInUse = 0;
|
||||
|
@ -335,6 +348,7 @@ public class ExternalTextureConverter implements TextureFrameProducer {
|
|||
}
|
||||
|
||||
public void setSurfaceTexture(SurfaceTexture texture, int width, int height) {
|
||||
hasReceivedFirstFrame = false;
|
||||
if (surfaceTexture != null) {
|
||||
surfaceTexture.setOnFrameAvailableListener(null);
|
||||
}
|
||||
|
@ -381,6 +395,10 @@ public class ExternalTextureConverter implements TextureFrameProducer {
|
|||
return surfaceTexture != null ? surfaceTexture : internalSurfaceTexture;
|
||||
}
|
||||
|
||||
public boolean getHasReceivedFirstFrame() {
|
||||
return hasReceivedFirstFrame;
|
||||
}
|
||||
|
||||
@Override
|
||||
public void onFrameAvailable(SurfaceTexture surfaceTexture) {
|
||||
handler.post(() -> renderNext(surfaceTexture));
|
||||
|
@ -427,6 +445,7 @@ public class ExternalTextureConverter implements TextureFrameProducer {
|
|||
// pending on the handler. When that happens, we should simply disregard the call.
|
||||
return;
|
||||
}
|
||||
hasReceivedFirstFrame = true;
|
||||
try {
|
||||
synchronized (consumers) {
|
||||
boolean frameUpdated = false;
|
||||
|
|
|
@ -69,7 +69,8 @@ objc_library(
|
|||
"-Wno-shorten-64-to-32",
|
||||
],
|
||||
sdk_frameworks = ["Accelerate"],
|
||||
visibility = ["//mediapipe/framework:mediapipe_internal"],
|
||||
# This build rule is public to allow external customers to build their own iOS apps.
|
||||
visibility = ["//visibility:public"],
|
||||
deps = [
|
||||
":CFHolder",
|
||||
":util",
|
||||
|
@ -124,7 +125,8 @@ objc_library(
|
|||
"CoreVideo",
|
||||
"Foundation",
|
||||
],
|
||||
visibility = ["//mediapipe/framework:mediapipe_internal"],
|
||||
# This build rule is public to allow external customers to build their own iOS apps.
|
||||
visibility = ["//visibility:public"],
|
||||
)
|
||||
|
||||
objc_library(
|
||||
|
@ -166,7 +168,8 @@ objc_library(
|
|||
"Foundation",
|
||||
"GLKit",
|
||||
],
|
||||
visibility = ["//mediapipe/framework:mediapipe_internal"],
|
||||
# This build rule is public to allow external customers to build their own iOS apps.
|
||||
visibility = ["//visibility:public"],
|
||||
deps = [
|
||||
":mediapipe_framework_ios",
|
||||
":mediapipe_gl_view_renderer",
|
||||
|
|
|
@ -23,7 +23,7 @@
|
|||
@property(nonatomic, getter=isAuthorized, readonly) BOOL authorized;
|
||||
|
||||
/// Session preset to use for capturing.
|
||||
@property(nonatomic) NSString *sessionPreset;
|
||||
@property(nonatomic, nullable) NSString *sessionPreset;
|
||||
|
||||
/// Which camera on an iOS device to use, assuming iOS device with more than one camera.
|
||||
@property(nonatomic) AVCaptureDevicePosition cameraPosition;
|
||||
|
|
|
@ -17,21 +17,21 @@ import os
|
|||
import shutil
|
||||
import urllib.request
|
||||
|
||||
_OSS_URL_PREFIX = 'https://github.com/google/mediapipe/raw/master/'
|
||||
_GCS_URL_PREFIX = 'https://storage.googleapis.com/mediapipe-assets/'
|
||||
|
||||
|
||||
def download_oss_model(model_path: str):
|
||||
"""Downloads the oss model from the MediaPipe GitHub repo if it doesn't exist in the package."""
|
||||
"""Downloads the oss model from Google Cloud Storage if it doesn't exist in the package."""
|
||||
|
||||
mp_root_path = os.sep.join(os.path.abspath(__file__).split(os.sep)[:-4])
|
||||
model_abspath = os.path.join(mp_root_path, model_path)
|
||||
if os.path.exists(model_abspath):
|
||||
return
|
||||
model_url = _OSS_URL_PREFIX + model_path
|
||||
model_url = _GCS_URL_PREFIX + model_path.split('/')[-1]
|
||||
print('Downloading model to ' + model_abspath)
|
||||
with urllib.request.urlopen(model_url) as response, open(model_abspath,
|
||||
'wb') as out_file:
|
||||
if response.code != 200:
|
||||
raise ConnectionError('Cannot download ' + model_path +
|
||||
' from the MediaPipe Github repo.')
|
||||
' from Google Cloud Storage.')
|
||||
shutil.copyfileobj(response, out_file)
|
||||
|
|
|
@ -25,7 +25,7 @@ message AudioClassifierOptions {
|
|||
extend mediapipe.CalculatorOptions {
|
||||
optional AudioClassifierOptions ext = 451755788;
|
||||
}
|
||||
// Base options for configuring Task library, such as specifying the TfLite
|
||||
// Base options for configuring MediaPipe Tasks, such as specifying the TfLite
|
||||
// model file with metadata, accelerator options, etc.
|
||||
optional core.proto.BaseOptions base_options = 1;
|
||||
|
||||
|
|
|
@ -43,3 +43,73 @@ cc_library(
|
|||
],
|
||||
alwayslink = 1,
|
||||
)
|
||||
|
||||
mediapipe_proto_library(
|
||||
name = "score_calibration_calculator_proto",
|
||||
srcs = ["score_calibration_calculator.proto"],
|
||||
deps = [
|
||||
"//mediapipe/framework:calculator_options_proto",
|
||||
"//mediapipe/framework:calculator_proto",
|
||||
],
|
||||
)
|
||||
|
||||
cc_library(
|
||||
name = "score_calibration_calculator",
|
||||
srcs = ["score_calibration_calculator.cc"],
|
||||
deps = [
|
||||
":score_calibration_calculator_cc_proto",
|
||||
"//mediapipe/framework:calculator_framework",
|
||||
"//mediapipe/framework/api2:node",
|
||||
"//mediapipe/framework/api2:port",
|
||||
"//mediapipe/framework/formats:tensor",
|
||||
"//mediapipe/framework/port:ret_check",
|
||||
"//mediapipe/tasks/cc:common",
|
||||
"@com_google_absl//absl/status",
|
||||
"@com_google_absl//absl/status:statusor",
|
||||
"@com_google_absl//absl/strings:str_format",
|
||||
],
|
||||
alwayslink = 1,
|
||||
)
|
||||
|
||||
cc_test(
|
||||
name = "score_calibration_calculator_test",
|
||||
srcs = ["score_calibration_calculator_test.cc"],
|
||||
deps = [
|
||||
":score_calibration_calculator",
|
||||
"//mediapipe/framework:calculator_framework",
|
||||
"//mediapipe/framework:calculator_runner",
|
||||
"//mediapipe/framework/formats:tensor",
|
||||
"//mediapipe/framework/port:gtest_main",
|
||||
"//mediapipe/framework/port:parse_text_proto",
|
||||
"@com_google_absl//absl/status",
|
||||
"@com_google_absl//absl/strings:str_format",
|
||||
],
|
||||
)
|
||||
|
||||
cc_library(
|
||||
name = "score_calibration_utils",
|
||||
srcs = ["score_calibration_utils.cc"],
|
||||
hdrs = ["score_calibration_utils.h"],
|
||||
deps = [
|
||||
":score_calibration_calculator_cc_proto",
|
||||
"//mediapipe/framework/port:status",
|
||||
"//mediapipe/tasks/cc:common",
|
||||
"//mediapipe/tasks/metadata:metadata_schema_cc",
|
||||
"@com_google_absl//absl/status",
|
||||
"@com_google_absl//absl/strings",
|
||||
"@com_google_absl//absl/strings:str_format",
|
||||
],
|
||||
)
|
||||
|
||||
cc_test(
|
||||
name = "score_calibration_utils_test",
|
||||
srcs = ["score_calibration_utils_test.cc"],
|
||||
deps = [
|
||||
":score_calibration_calculator_cc_proto",
|
||||
":score_calibration_utils",
|
||||
"//mediapipe/framework/port:gtest_main",
|
||||
"//mediapipe/framework/port:parse_text_proto",
|
||||
"//mediapipe/tasks/metadata:metadata_schema_cc",
|
||||
"@com_google_absl//absl/strings",
|
||||
],
|
||||
)
|
||||
|
|
|
@ -0,0 +1,259 @@
|
|||
// Copyright 2022 The MediaPipe Authors.
|
||||
//
|
||||
// Licensed under the Apache License, Version 2.0 (the "License");
|
||||
// you may not use this file except in compliance with the License.
|
||||
// You may obtain a copy of the License at
|
||||
//
|
||||
// http://www.apache.org/licenses/LICENSE-2.0
|
||||
//
|
||||
// Unless required by applicable law or agreed to in writing, software
|
||||
// distributed under the License is distributed on an "AS IS" BASIS,
|
||||
// WITHOUT WARRANTIES OR CONDITIONS OF ANY KIND, either express or implied.
|
||||
// See the License for the specific language governing permissions and
|
||||
// limitations under the License.
|
||||
|
||||
#include <functional>
|
||||
#include <memory>
|
||||
#include <utility>
|
||||
#include <vector>
|
||||
|
||||
#include "absl/status/status.h"
|
||||
#include "absl/status/statusor.h"
|
||||
#include "absl/strings/str_format.h"
|
||||
#include "mediapipe/framework/api2/node.h"
|
||||
#include "mediapipe/framework/api2/port.h"
|
||||
#include "mediapipe/framework/calculator_framework.h"
|
||||
#include "mediapipe/framework/formats/tensor.h"
|
||||
#include "mediapipe/framework/port/ret_check.h"
|
||||
#include "mediapipe/tasks/cc/common.h"
|
||||
#include "mediapipe/tasks/cc/components/calculators/score_calibration_calculator.pb.h"
|
||||
|
||||
namespace mediapipe {
|
||||
namespace api2 {
|
||||
|
||||
using ::absl::StatusCode;
|
||||
using ::mediapipe::tasks::CreateStatusWithPayload;
|
||||
using ::mediapipe::tasks::MediaPipeTasksStatus;
|
||||
using ::mediapipe::tasks::ScoreCalibrationCalculatorOptions;
|
||||
|
||||
namespace {
|
||||
// Used to prevent log(<=0.0) in ClampedLog() calls.
|
||||
constexpr float kLogScoreMinimum = 1e-16;
|
||||
|
||||
// Returns the following, depending on x:
|
||||
// x => threshold: log(x)
|
||||
// x < threshold: 2 * log(thresh) - log(2 * thresh - x)
|
||||
// This form (a) is anti-symmetric about the threshold and (b) has continuous
|
||||
// value and first derivative. This is done to prevent taking the log of values
|
||||
// close to 0 which can lead to floating point errors and is better than simple
|
||||
// clamping since it preserves order for scores less than the threshold.
|
||||
float ClampedLog(float x, float threshold) {
|
||||
if (x < threshold) {
|
||||
return 2.0 * std::log(static_cast<double>(threshold)) -
|
||||
log(2.0 * threshold - x);
|
||||
}
|
||||
return std::log(static_cast<double>(x));
|
||||
}
|
||||
} // namespace
|
||||
|
||||
// Applies score calibration to a tensor of score predictions, typically applied
|
||||
// to the output of a classification or object detection model.
|
||||
//
|
||||
// See corresponding options for more details on the score calibration
|
||||
// parameters and formula.
|
||||
//
|
||||
// Inputs:
|
||||
// SCORES - std::vector<Tensor>
|
||||
// A vector containing a single Tensor `x` of type kFloat32, representing
|
||||
// the scores to calibrate. By default (i.e. if INDICES is not connected),
|
||||
// x[i] will be calibrated using the sigmoid provided at index i in the
|
||||
// options.
|
||||
// INDICES - std::vector<Tensor> @Optional
|
||||
// An optional vector containing a single Tensor `y` of type kFloat32 and
|
||||
// same size as `x`. If provided, x[i] will be calibrated using the sigmoid
|
||||
// provided at index y[i] (casted as an integer) in the options. `x` and `y`
|
||||
// must contain the same number of elements. Typically used for object
|
||||
// detection models.
|
||||
//
|
||||
// Outputs:
|
||||
// CALIBRATED_SCORES - std::vector<Tensor>
|
||||
// A vector containing a single Tensor of type kFloat32 and of the same size
|
||||
// as the input tensors. Contains the output calibrated scores.
|
||||
class ScoreCalibrationCalculator : public Node {
|
||||
public:
|
||||
static constexpr Input<std::vector<Tensor>> kScoresIn{"SCORES"};
|
||||
static constexpr Input<std::vector<Tensor>>::Optional kIndicesIn{"INDICES"};
|
||||
static constexpr Output<std::vector<Tensor>> kScoresOut{"CALIBRATED_SCORES"};
|
||||
MEDIAPIPE_NODE_CONTRACT(kScoresIn, kIndicesIn, kScoresOut);
|
||||
|
||||
absl::Status Open(CalculatorContext* cc) override;
|
||||
absl::Status Process(CalculatorContext* cc) override;
|
||||
|
||||
private:
|
||||
ScoreCalibrationCalculatorOptions options_;
|
||||
std::function<float(float)> score_transformation_;
|
||||
|
||||
// Computes the calibrated score for the provided index. Does not check for
|
||||
// out-of-bounds index.
|
||||
float ComputeCalibratedScore(int index, float score);
|
||||
// Same as above, but does check for out-of-bounds index.
|
||||
absl::StatusOr<float> SafeComputeCalibratedScore(int index, float score);
|
||||
};
|
||||
|
||||
absl::Status ScoreCalibrationCalculator::Open(CalculatorContext* cc) {
|
||||
options_ = cc->Options<ScoreCalibrationCalculatorOptions>();
|
||||
// Sanity checks.
|
||||
if (options_.sigmoids_size() == 0) {
|
||||
return CreateStatusWithPayload(StatusCode::kInvalidArgument,
|
||||
"Expected at least one sigmoid, found none.",
|
||||
MediaPipeTasksStatus::kInvalidArgumentError);
|
||||
}
|
||||
for (const auto& sigmoid : options_.sigmoids()) {
|
||||
if (sigmoid.has_scale() && sigmoid.scale() < 0.0) {
|
||||
return CreateStatusWithPayload(
|
||||
StatusCode::kInvalidArgument,
|
||||
absl::StrFormat("The scale parameter of the sigmoids must be "
|
||||
"positive, found %f.",
|
||||
sigmoid.scale()),
|
||||
MediaPipeTasksStatus::kInvalidArgumentError);
|
||||
}
|
||||
}
|
||||
// Set score transformation function once and for all.
|
||||
switch (options_.score_transformation()) {
|
||||
case tasks::ScoreCalibrationCalculatorOptions::IDENTITY:
|
||||
score_transformation_ = [](float x) { return x; };
|
||||
break;
|
||||
case tasks::ScoreCalibrationCalculatorOptions::LOG:
|
||||
score_transformation_ = [](float x) {
|
||||
return ClampedLog(x, kLogScoreMinimum);
|
||||
};
|
||||
break;
|
||||
case tasks::ScoreCalibrationCalculatorOptions::INVERSE_LOGISTIC:
|
||||
score_transformation_ = [](float x) {
|
||||
return (ClampedLog(x, kLogScoreMinimum) -
|
||||
ClampedLog(1.0 - x, kLogScoreMinimum));
|
||||
};
|
||||
break;
|
||||
default:
|
||||
return CreateStatusWithPayload(
|
||||
StatusCode::kInvalidArgument,
|
||||
absl::StrFormat(
|
||||
"Unsupported ScoreTransformation type: %s",
|
||||
ScoreCalibrationCalculatorOptions::ScoreTransformation_Name(
|
||||
options_.score_transformation())),
|
||||
MediaPipeTasksStatus::kInvalidArgumentError);
|
||||
}
|
||||
return absl::OkStatus();
|
||||
}
|
||||
|
||||
absl::Status ScoreCalibrationCalculator::Process(CalculatorContext* cc) {
|
||||
RET_CHECK_EQ(kScoresIn(cc)->size(), 1);
|
||||
const auto& scores = (*kScoresIn(cc))[0];
|
||||
RET_CHECK(scores.element_type() == Tensor::ElementType::kFloat32);
|
||||
auto scores_view = scores.GetCpuReadView();
|
||||
const float* raw_scores = scores_view.buffer<float>();
|
||||
int num_scores = scores.shape().num_elements();
|
||||
|
||||
auto output_tensors = std::make_unique<std::vector<Tensor>>();
|
||||
output_tensors->reserve(1);
|
||||
output_tensors->emplace_back(scores.element_type(), scores.shape());
|
||||
auto calibrated_scores = &output_tensors->back();
|
||||
auto calibrated_scores_view = calibrated_scores->GetCpuWriteView();
|
||||
float* raw_calibrated_scores = calibrated_scores_view.buffer<float>();
|
||||
|
||||
if (kIndicesIn(cc).IsConnected()) {
|
||||
RET_CHECK_EQ(kIndicesIn(cc)->size(), 1);
|
||||
const auto& indices = (*kIndicesIn(cc))[0];
|
||||
RET_CHECK(indices.element_type() == Tensor::ElementType::kFloat32);
|
||||
if (num_scores != indices.shape().num_elements()) {
|
||||
return CreateStatusWithPayload(
|
||||
StatusCode::kInvalidArgument,
|
||||
absl::StrFormat("Mismatch between number of elements in the input "
|
||||
"scores tensor (%d) and indices tensor (%d).",
|
||||
num_scores, indices.shape().num_elements()),
|
||||
MediaPipeTasksStatus::kMetadataInconsistencyError);
|
||||
}
|
||||
auto indices_view = indices.GetCpuReadView();
|
||||
const float* raw_indices = indices_view.buffer<float>();
|
||||
for (int i = 0; i < num_scores; ++i) {
|
||||
// Use the "safe" flavor as we need to check that the externally provided
|
||||
// indices are not out-of-bounds.
|
||||
ASSIGN_OR_RETURN(raw_calibrated_scores[i],
|
||||
SafeComputeCalibratedScore(
|
||||
static_cast<int>(raw_indices[i]), raw_scores[i]));
|
||||
}
|
||||
} else {
|
||||
if (num_scores != options_.sigmoids_size()) {
|
||||
return CreateStatusWithPayload(
|
||||
StatusCode::kInvalidArgument,
|
||||
absl::StrFormat("Mismatch between number of sigmoids (%d) and number "
|
||||
"of elements in the input scores tensor (%d).",
|
||||
options_.sigmoids_size(), num_scores),
|
||||
MediaPipeTasksStatus::kMetadataInconsistencyError);
|
||||
}
|
||||
for (int i = 0; i < num_scores; ++i) {
|
||||
// Use the "unsafe" flavor as we have already checked for out-of-bounds
|
||||
// issues.
|
||||
raw_calibrated_scores[i] = ComputeCalibratedScore(i, raw_scores[i]);
|
||||
}
|
||||
}
|
||||
kScoresOut(cc).Send(std::move(output_tensors));
|
||||
return absl::OkStatus();
|
||||
}
|
||||
|
||||
float ScoreCalibrationCalculator::ComputeCalibratedScore(int index,
|
||||
float score) {
|
||||
const auto& sigmoid = options_.sigmoids(index);
|
||||
|
||||
bool is_empty =
|
||||
!sigmoid.has_scale() || !sigmoid.has_offset() || !sigmoid.has_slope();
|
||||
bool is_below_min_score =
|
||||
sigmoid.has_min_score() && score < sigmoid.min_score();
|
||||
if (is_empty || is_below_min_score) {
|
||||
return options_.default_score();
|
||||
}
|
||||
|
||||
float transformed_score = score_transformation_(score);
|
||||
float scale_shifted_score =
|
||||
transformed_score * sigmoid.slope() + sigmoid.offset();
|
||||
// For numerical stability use 1 / (1+exp(-x)) when scale_shifted_score >= 0
|
||||
// and exp(x) / (1+exp(x)) when scale_shifted_score < 0.
|
||||
float calibrated_score;
|
||||
if (scale_shifted_score >= 0.0) {
|
||||
calibrated_score =
|
||||
sigmoid.scale() /
|
||||
(1.0 + std::exp(static_cast<double>(-scale_shifted_score)));
|
||||
} else {
|
||||
float score_exp = std::exp(static_cast<double>(scale_shifted_score));
|
||||
calibrated_score = sigmoid.scale() * score_exp / (1.0 + score_exp);
|
||||
}
|
||||
// Scale is non-negative (checked in SigmoidFromLabelAndLine),
|
||||
// thus calibrated_score should be in the range of [0, scale]. However, due to
|
||||
// numberical stability issue, it may fall out of the boundary. Cap the value
|
||||
// to [0, scale] instead.
|
||||
return std::max(std::min(calibrated_score, sigmoid.scale()), 0.0f);
|
||||
}
|
||||
|
||||
absl::StatusOr<float> ScoreCalibrationCalculator::SafeComputeCalibratedScore(
|
||||
int index, float score) {
|
||||
if (index < 0) {
|
||||
return CreateStatusWithPayload(
|
||||
StatusCode::kInvalidArgument,
|
||||
absl::StrFormat("Expected positive indices, found %d.", index),
|
||||
MediaPipeTasksStatus::kInvalidArgumentError);
|
||||
}
|
||||
if (index > options_.sigmoids_size()) {
|
||||
return CreateStatusWithPayload(
|
||||
StatusCode::kInvalidArgument,
|
||||
absl::StrFormat("Unable to get score calibration parameters for index "
|
||||
"%d : only %d sigmoids were provided.",
|
||||
index, options_.sigmoids_size()),
|
||||
MediaPipeTasksStatus::kMetadataInconsistencyError);
|
||||
}
|
||||
return ComputeCalibratedScore(index, score);
|
||||
}
|
||||
|
||||
MEDIAPIPE_REGISTER_NODE(ScoreCalibrationCalculator);
|
||||
|
||||
} // namespace api2
|
||||
} // namespace mediapipe
|
|
@ -0,0 +1,67 @@
|
|||
/* Copyright 2022 The MediaPipe Authors. All Rights Reserved.
|
||||
|
||||
Licensed under the Apache License, Version 2.0 (the "License");
|
||||
you may not use this file except in compliance with the License.
|
||||
You may obtain a copy of the License at
|
||||
|
||||
http://www.apache.org/licenses/LICENSE-2.0
|
||||
|
||||
Unless required by applicable law or agreed to in writing, software
|
||||
distributed under the License is distributed on an "AS IS" BASIS,
|
||||
WITHOUT WARRANTIES OR CONDITIONS OF ANY KIND, either express or implied.
|
||||
See the License for the specific language governing permissions and
|
||||
limitations under the License.
|
||||
==============================================================================*/
|
||||
|
||||
syntax = "proto2";
|
||||
|
||||
package mediapipe.tasks;
|
||||
|
||||
import "mediapipe/framework/calculator.proto";
|
||||
|
||||
message ScoreCalibrationCalculatorOptions {
|
||||
extend mediapipe.CalculatorOptions {
|
||||
optional ScoreCalibrationCalculatorOptions ext = 470204318;
|
||||
}
|
||||
|
||||
// Score calibration parameters for one individual category. The formula used
|
||||
// to transform the uncalibrated score `x` is:
|
||||
// * `f(x) = scale / (1 + e^-(slope * g(x) + offset))` if `x > min_score` or
|
||||
// if no min_score has been specified,
|
||||
// * `f(x) = default_score` otherwise or if no scale, slope or offset have
|
||||
// been specified.
|
||||
//
|
||||
// Where:
|
||||
// * scale must be positive,
|
||||
// * g(x) is a global (i.e. category independent) transform specified using
|
||||
// the score_transformation field,
|
||||
// * default_score is a global parameter defined below.
|
||||
//
|
||||
// There should be exactly one sigmoid per number of supported output
|
||||
// categories in the model, with either:
|
||||
// * no fields set,
|
||||
// * scale, slope and offset set,
|
||||
// * all fields set.
|
||||
message Sigmoid {
|
||||
optional float scale = 1;
|
||||
optional float slope = 2;
|
||||
optional float offset = 3;
|
||||
optional float min_score = 4;
|
||||
}
|
||||
repeated Sigmoid sigmoids = 1;
|
||||
|
||||
// Score transformation that defines the `g(x)` function in the above formula.
|
||||
enum ScoreTransformation {
|
||||
UNSPECIFIED = 0;
|
||||
// g(x) = x.
|
||||
IDENTITY = 1;
|
||||
// g(x) = log(x).
|
||||
LOG = 2;
|
||||
// g(x) = log(x) - log(1 - x).
|
||||
INVERSE_LOGISTIC = 3;
|
||||
}
|
||||
optional ScoreTransformation score_transformation = 2 [default = IDENTITY];
|
||||
|
||||
// Default score.
|
||||
optional float default_score = 3;
|
||||
}
|
|
@ -0,0 +1,309 @@
|
|||
// Copyright 2022 The MediaPipe Authors.
|
||||
//
|
||||
// Licensed under the Apache License, Version 2.0 (the "License");
|
||||
// you may not use this file except in compliance with the License.
|
||||
// You may obtain a copy of the License at
|
||||
//
|
||||
// http://www.apache.org/licenses/LICENSE-2.0
|
||||
//
|
||||
// Unless required by applicable law or agreed to in writing, software
|
||||
// distributed under the License is distributed on an "AS IS" BASIS,
|
||||
// WITHOUT WARRANTIES OR CONDITIONS OF ANY KIND, either express or implied.
|
||||
// See the License for the specific language governing permissions and
|
||||
// limitations under the License.
|
||||
|
||||
#include <cstdint>
|
||||
#include <memory>
|
||||
#include <optional>
|
||||
#include <vector>
|
||||
|
||||
#include "absl/status/status.h"
|
||||
#include "absl/strings/str_format.h"
|
||||
#include "mediapipe/framework/calculator_framework.h"
|
||||
#include "mediapipe/framework/calculator_runner.h"
|
||||
#include "mediapipe/framework/formats/tensor.h"
|
||||
#include "mediapipe/framework/port/gmock.h"
|
||||
#include "mediapipe/framework/port/gtest.h"
|
||||
#include "mediapipe/framework/port/parse_text_proto.h"
|
||||
#include "mediapipe/framework/port/status_matchers.h"
|
||||
|
||||
namespace mediapipe {
|
||||
namespace {
|
||||
|
||||
using ::mediapipe::ParseTextProtoOrDie;
|
||||
using ::testing::HasSubstr;
|
||||
using ::testing::TestParamInfo;
|
||||
using ::testing::TestWithParam;
|
||||
using ::testing::Values;
|
||||
using Node = ::mediapipe::CalculatorGraphConfig::Node;
|
||||
|
||||
// Builds the graph and feeds inputs.
|
||||
void BuildGraph(CalculatorRunner* runner, std::vector<float> scores,
|
||||
std::optional<std::vector<int>> indices = std::nullopt) {
|
||||
auto scores_tensors = std::make_unique<std::vector<Tensor>>();
|
||||
scores_tensors->emplace_back(
|
||||
Tensor::ElementType::kFloat32,
|
||||
Tensor::Shape{1, static_cast<int>(scores.size())});
|
||||
auto scores_view = scores_tensors->back().GetCpuWriteView();
|
||||
float* scores_buffer = scores_view.buffer<float>();
|
||||
ASSERT_NE(scores_buffer, nullptr);
|
||||
for (int i = 0; i < scores.size(); ++i) {
|
||||
scores_buffer[i] = scores[i];
|
||||
}
|
||||
auto& input_scores_packets = runner->MutableInputs()->Tag("SCORES").packets;
|
||||
input_scores_packets.push_back(
|
||||
mediapipe::Adopt(scores_tensors.release()).At(mediapipe::Timestamp(0)));
|
||||
|
||||
if (indices.has_value()) {
|
||||
auto indices_tensors = std::make_unique<std::vector<Tensor>>();
|
||||
indices_tensors->emplace_back(
|
||||
Tensor::ElementType::kFloat32,
|
||||
Tensor::Shape{1, static_cast<int>(indices->size())});
|
||||
auto indices_view = indices_tensors->back().GetCpuWriteView();
|
||||
float* indices_buffer = indices_view.buffer<float>();
|
||||
ASSERT_NE(indices_buffer, nullptr);
|
||||
for (int i = 0; i < indices->size(); ++i) {
|
||||
indices_buffer[i] = static_cast<float>((*indices)[i]);
|
||||
}
|
||||
auto& input_indices_packets =
|
||||
runner->MutableInputs()->Tag("INDICES").packets;
|
||||
input_indices_packets.push_back(mediapipe::Adopt(indices_tensors.release())
|
||||
.At(mediapipe::Timestamp(0)));
|
||||
}
|
||||
}
|
||||
|
||||
// Compares the provided tensor contents with the expected values.
|
||||
void ValidateResult(const Tensor& actual, const std::vector<float>& expected) {
|
||||
EXPECT_EQ(actual.element_type(), Tensor::ElementType::kFloat32);
|
||||
EXPECT_EQ(expected.size(), actual.shape().num_elements());
|
||||
auto view = actual.GetCpuReadView();
|
||||
auto buffer = view.buffer<float>();
|
||||
for (int i = 0; i < expected.size(); ++i) {
|
||||
EXPECT_FLOAT_EQ(expected[i], buffer[i]);
|
||||
}
|
||||
}
|
||||
|
||||
TEST(ScoreCalibrationCalculatorTest, FailsWithNoSigmoid) {
|
||||
CalculatorRunner runner(ParseTextProtoOrDie<Node>(R"pb(
|
||||
calculator: "ScoreCalibrationCalculator"
|
||||
input_stream: "SCORES:scores"
|
||||
output_stream: "CALIBRATED_SCORES:calibrated_scores"
|
||||
options {
|
||||
[mediapipe.tasks.ScoreCalibrationCalculatorOptions.ext] {}
|
||||
}
|
||||
)pb"));
|
||||
|
||||
BuildGraph(&runner, {0.5, 0.5, 0.5});
|
||||
auto status = runner.Run();
|
||||
|
||||
EXPECT_EQ(status.code(), absl::StatusCode::kInvalidArgument);
|
||||
EXPECT_THAT(status.message(),
|
||||
HasSubstr("Expected at least one sigmoid, found none"));
|
||||
}
|
||||
|
||||
TEST(ScoreCalibrationCalculatorTest, FailsWithNegativeScale) {
|
||||
CalculatorRunner runner(ParseTextProtoOrDie<Node>(R"pb(
|
||||
calculator: "ScoreCalibrationCalculator"
|
||||
input_stream: "SCORES:scores"
|
||||
output_stream: "CALIBRATED_SCORES:calibrated_scores"
|
||||
options {
|
||||
[mediapipe.tasks.ScoreCalibrationCalculatorOptions.ext] {
|
||||
sigmoids { slope: 1 offset: 1 scale: -1 }
|
||||
}
|
||||
}
|
||||
)pb"));
|
||||
|
||||
BuildGraph(&runner, {0.5, 0.5, 0.5});
|
||||
auto status = runner.Run();
|
||||
|
||||
EXPECT_EQ(status.code(), absl::StatusCode::kInvalidArgument);
|
||||
EXPECT_THAT(
|
||||
status.message(),
|
||||
HasSubstr("The scale parameter of the sigmoids must be positive"));
|
||||
}
|
||||
|
||||
TEST(ScoreCalibrationCalculatorTest, FailsWithUnspecifiedTransformation) {
|
||||
CalculatorRunner runner(ParseTextProtoOrDie<Node>(R"pb(
|
||||
calculator: "ScoreCalibrationCalculator"
|
||||
input_stream: "SCORES:scores"
|
||||
output_stream: "CALIBRATED_SCORES:calibrated_scores"
|
||||
options {
|
||||
[mediapipe.tasks.ScoreCalibrationCalculatorOptions.ext] {
|
||||
sigmoids { slope: 1 offset: 1 scale: 1 }
|
||||
score_transformation: UNSPECIFIED
|
||||
}
|
||||
}
|
||||
)pb"));
|
||||
|
||||
BuildGraph(&runner, {0.5, 0.5, 0.5});
|
||||
auto status = runner.Run();
|
||||
|
||||
EXPECT_EQ(status.code(), absl::StatusCode::kInvalidArgument);
|
||||
EXPECT_THAT(status.message(),
|
||||
HasSubstr("Unsupported ScoreTransformation type"));
|
||||
}
|
||||
|
||||
// Struct holding the parameters for parameterized tests below.
|
||||
struct CalibrationTestParams {
|
||||
// The score transformation to apply.
|
||||
std::string score_transformation;
|
||||
// The expected results.
|
||||
std::vector<float> expected_results;
|
||||
};
|
||||
|
||||
class CalibrationWithoutIndicesTest
|
||||
: public TestWithParam<CalibrationTestParams> {};
|
||||
|
||||
TEST_P(CalibrationWithoutIndicesTest, Succeeds) {
|
||||
CalculatorRunner runner(ParseTextProtoOrDie<Node>(absl::StrFormat(
|
||||
R"pb(
|
||||
calculator: "ScoreCalibrationCalculator"
|
||||
input_stream: "SCORES:scores"
|
||||
output_stream: "CALIBRATED_SCORES:calibrated_scores"
|
||||
options {
|
||||
[mediapipe.tasks.ScoreCalibrationCalculatorOptions.ext] {
|
||||
sigmoids { scale: 0.9 slope: 0.5 offset: 0.1 }
|
||||
sigmoids { scale: 0.9 slope: 0.5 offset: 0.1 min_score: 0.3 }
|
||||
sigmoids { scale: 0.9 slope: 0.5 offset: 0.1 min_score: 0.6 }
|
||||
sigmoids {}
|
||||
score_transformation: %s
|
||||
default_score: 0.2
|
||||
}
|
||||
}
|
||||
)pb",
|
||||
GetParam().score_transformation)));
|
||||
|
||||
BuildGraph(&runner, {0.2, 0.3, 0.4, 0.5});
|
||||
MP_ASSERT_OK(runner.Run());
|
||||
const Tensor& results = runner.Outputs()
|
||||
.Get("CALIBRATED_SCORES", 0)
|
||||
.packets[0]
|
||||
.Get<std::vector<Tensor>>()[0];
|
||||
|
||||
ValidateResult(results, GetParam().expected_results);
|
||||
}
|
||||
|
||||
INSTANTIATE_TEST_SUITE_P(
|
||||
ScoreCalibrationCalculatorTest, CalibrationWithoutIndicesTest,
|
||||
Values(CalibrationTestParams{.score_transformation = "IDENTITY",
|
||||
.expected_results = {0.4948505976,
|
||||
0.5059588508, 0.2, 0.2}},
|
||||
CalibrationTestParams{
|
||||
.score_transformation = "LOG",
|
||||
.expected_results = {0.2976901255, 0.3393665735, 0.2, 0.2}},
|
||||
CalibrationTestParams{
|
||||
.score_transformation = "INVERSE_LOGISTIC",
|
||||
.expected_results = {0.3203217641, 0.3778080605, 0.2, 0.2}}),
|
||||
[](const TestParamInfo<CalibrationWithoutIndicesTest::ParamType>& info) {
|
||||
return info.param.score_transformation;
|
||||
});
|
||||
|
||||
TEST(ScoreCalibrationCalculatorTest, FailsWithMissingSigmoids) {
|
||||
CalculatorRunner runner(ParseTextProtoOrDie<Node>(R"pb(
|
||||
calculator: "ScoreCalibrationCalculator"
|
||||
input_stream: "SCORES:scores"
|
||||
output_stream: "CALIBRATED_SCORES:calibrated_scores"
|
||||
options {
|
||||
[mediapipe.tasks.ScoreCalibrationCalculatorOptions.ext] {
|
||||
sigmoids { scale: 0.9 slope: 0.5 offset: 0.1 }
|
||||
sigmoids { scale: 0.9 slope: 0.5 offset: 0.1 min_score: 0.3 }
|
||||
sigmoids { scale: 0.9 slope: 0.5 offset: 0.1 min_score: 0.6 }
|
||||
sigmoids {}
|
||||
score_transformation: LOG
|
||||
default_score: 0.2
|
||||
}
|
||||
}
|
||||
)pb"));
|
||||
|
||||
BuildGraph(&runner, {0.2, 0.3, 0.4, 0.5, 0.6});
|
||||
auto status = runner.Run();
|
||||
|
||||
EXPECT_EQ(status.code(), absl::StatusCode::kInvalidArgument);
|
||||
EXPECT_THAT(status.message(),
|
||||
HasSubstr("Mismatch between number of sigmoids"));
|
||||
}
|
||||
|
||||
TEST(ScoreCalibrationCalculatorTest, SucceedsWithIndices) {
|
||||
CalculatorRunner runner(ParseTextProtoOrDie<Node>(R"pb(
|
||||
calculator: "ScoreCalibrationCalculator"
|
||||
input_stream: "SCORES:scores"
|
||||
input_stream: "INDICES:indices"
|
||||
output_stream: "CALIBRATED_SCORES:calibrated_scores"
|
||||
options {
|
||||
[mediapipe.tasks.ScoreCalibrationCalculatorOptions.ext] {
|
||||
sigmoids { scale: 0.9 slope: 0.5 offset: 0.1 }
|
||||
sigmoids { scale: 0.9 slope: 0.5 offset: 0.1 min_score: 0.3 }
|
||||
sigmoids { scale: 0.9 slope: 0.5 offset: 0.1 min_score: 0.6 }
|
||||
sigmoids {}
|
||||
score_transformation: IDENTITY
|
||||
default_score: 0.2
|
||||
}
|
||||
}
|
||||
)pb"));
|
||||
std::vector<int> indices = {1, 2, 3, 0};
|
||||
|
||||
BuildGraph(&runner, {0.3, 0.4, 0.5, 0.2}, indices);
|
||||
MP_ASSERT_OK(runner.Run());
|
||||
const Tensor& results = runner.Outputs()
|
||||
.Get("CALIBRATED_SCORES", 0)
|
||||
.packets[0]
|
||||
.Get<std::vector<Tensor>>()[0];
|
||||
ValidateResult(results, {0.5059588508, 0.2, 0.2, 0.4948505976});
|
||||
}
|
||||
|
||||
TEST(ScoreCalibrationCalculatorTest, FailsWithNegativeIndex) {
|
||||
CalculatorRunner runner(ParseTextProtoOrDie<Node>(R"pb(
|
||||
calculator: "ScoreCalibrationCalculator"
|
||||
input_stream: "SCORES:scores"
|
||||
input_stream: "INDICES:indices"
|
||||
output_stream: "CALIBRATED_SCORES:calibrated_scores"
|
||||
options {
|
||||
[mediapipe.tasks.ScoreCalibrationCalculatorOptions.ext] {
|
||||
sigmoids { scale: 0.9 slope: 0.5 offset: 0.1 }
|
||||
sigmoids { scale: 0.9 slope: 0.5 offset: 0.1 min_score: 0.3 }
|
||||
sigmoids { scale: 0.9 slope: 0.5 offset: 0.1 min_score: 0.6 }
|
||||
sigmoids {}
|
||||
score_transformation: IDENTITY
|
||||
default_score: 0.2
|
||||
}
|
||||
}
|
||||
)pb"));
|
||||
std::vector<int> indices = {0, 1, 2, -1};
|
||||
|
||||
BuildGraph(&runner, {0.2, 0.3, 0.4, 0.5}, indices);
|
||||
auto status = runner.Run();
|
||||
|
||||
EXPECT_EQ(status.code(), absl::StatusCode::kInvalidArgument);
|
||||
EXPECT_THAT(status.message(), HasSubstr("Expected positive indices"));
|
||||
}
|
||||
|
||||
TEST(ScoreCalibrationCalculatorTest, FailsWithOutOfBoundsIndex) {
|
||||
CalculatorRunner runner(ParseTextProtoOrDie<Node>(R"pb(
|
||||
calculator: "ScoreCalibrationCalculator"
|
||||
input_stream: "SCORES:scores"
|
||||
input_stream: "INDICES:indices"
|
||||
output_stream: "CALIBRATED_SCORES:calibrated_scores"
|
||||
options {
|
||||
[mediapipe.tasks.ScoreCalibrationCalculatorOptions.ext] {
|
||||
sigmoids { scale: 0.9 slope: 0.5 offset: 0.1 }
|
||||
sigmoids { scale: 0.9 slope: 0.5 offset: 0.1 min_score: 0.3 }
|
||||
sigmoids { scale: 0.9 slope: 0.5 offset: 0.1 min_score: 0.6 }
|
||||
sigmoids {}
|
||||
score_transformation: IDENTITY
|
||||
default_score: 0.2
|
||||
}
|
||||
}
|
||||
)pb"));
|
||||
std::vector<int> indices = {0, 1, 5, 3};
|
||||
|
||||
BuildGraph(&runner, {0.2, 0.3, 0.4, 0.5}, indices);
|
||||
auto status = runner.Run();
|
||||
|
||||
EXPECT_EQ(status.code(), absl::StatusCode::kInvalidArgument);
|
||||
EXPECT_THAT(
|
||||
status.message(),
|
||||
HasSubstr("Unable to get score calibration parameters for index"));
|
||||
}
|
||||
|
||||
} // namespace
|
||||
} // namespace mediapipe
|
|
@ -0,0 +1,115 @@
|
|||
// Copyright 2022 The MediaPipe Authors.
|
||||
//
|
||||
// Licensed under the Apache License, Version 2.0 (the "License");
|
||||
// you may not use this file except in compliance with the License.
|
||||
// You may obtain a copy of the License at
|
||||
//
|
||||
// http://www.apache.org/licenses/LICENSE-2.0
|
||||
//
|
||||
// Unless required by applicable law or agreed to in writing, software
|
||||
// distributed under the License is distributed on an "AS IS" BASIS,
|
||||
// WITHOUT WARRANTIES OR CONDITIONS OF ANY KIND, either express or implied.
|
||||
// See the License for the specific language governing permissions and
|
||||
// limitations under the License.
|
||||
|
||||
#include "mediapipe/tasks/cc/components/calculators/score_calibration_utils.h"
|
||||
|
||||
#include <vector>
|
||||
|
||||
#include "absl/status/status.h"
|
||||
#include "absl/strings/numbers.h"
|
||||
#include "absl/strings/str_format.h"
|
||||
#include "absl/strings/str_split.h"
|
||||
#include "absl/strings/string_view.h"
|
||||
#include "mediapipe/framework/port/status_macros.h"
|
||||
#include "mediapipe/tasks/cc/common.h"
|
||||
#include "mediapipe/tasks/cc/components/calculators/score_calibration_calculator.pb.h"
|
||||
#include "mediapipe/tasks/metadata/metadata_schema_generated.h"
|
||||
|
||||
namespace mediapipe {
|
||||
namespace tasks {
|
||||
|
||||
namespace {
|
||||
// Converts ScoreTransformation type from TFLite Metadata to calculator options.
|
||||
ScoreCalibrationCalculatorOptions::ScoreTransformation
|
||||
ConvertScoreTransformationType(tflite::ScoreTransformationType type) {
|
||||
switch (type) {
|
||||
case tflite::ScoreTransformationType_IDENTITY:
|
||||
return ScoreCalibrationCalculatorOptions::IDENTITY;
|
||||
case tflite::ScoreTransformationType_LOG:
|
||||
return ScoreCalibrationCalculatorOptions::LOG;
|
||||
case tflite::ScoreTransformationType_INVERSE_LOGISTIC:
|
||||
return ScoreCalibrationCalculatorOptions::INVERSE_LOGISTIC;
|
||||
}
|
||||
}
|
||||
|
||||
// Parses a single line of the score calibration file into the provided sigmoid.
|
||||
absl::Status FillSigmoidFromLine(
|
||||
absl::string_view line,
|
||||
ScoreCalibrationCalculatorOptions::Sigmoid* sigmoid) {
|
||||
if (line.empty()) {
|
||||
return absl::OkStatus();
|
||||
}
|
||||
std::vector<absl::string_view> str_params = absl::StrSplit(line, ',');
|
||||
if (str_params.size() != 3 && str_params.size() != 4) {
|
||||
return CreateStatusWithPayload(
|
||||
absl::StatusCode::kInvalidArgument,
|
||||
absl::StrFormat("Expected 3 or 4 parameters per line in score "
|
||||
"calibration file, got %d.",
|
||||
str_params.size()),
|
||||
MediaPipeTasksStatus::kMetadataMalformedScoreCalibrationError);
|
||||
}
|
||||
std::vector<float> params(str_params.size());
|
||||
for (int i = 0; i < str_params.size(); ++i) {
|
||||
if (!absl::SimpleAtof(str_params[i], ¶ms[i])) {
|
||||
return CreateStatusWithPayload(
|
||||
absl::StatusCode::kInvalidArgument,
|
||||
absl::StrFormat(
|
||||
"Could not parse score calibration parameter as float: %s.",
|
||||
str_params[i]),
|
||||
MediaPipeTasksStatus::kMetadataMalformedScoreCalibrationError);
|
||||
}
|
||||
}
|
||||
if (params[0] < 0) {
|
||||
return CreateStatusWithPayload(
|
||||
absl::StatusCode::kInvalidArgument,
|
||||
absl::StrFormat(
|
||||
"The scale parameter of the sigmoids must be positive, found %f.",
|
||||
params[0]),
|
||||
MediaPipeTasksStatus::kMetadataMalformedScoreCalibrationError);
|
||||
}
|
||||
sigmoid->set_scale(params[0]);
|
||||
sigmoid->set_slope(params[1]);
|
||||
sigmoid->set_offset(params[2]);
|
||||
if (params.size() == 4) {
|
||||
sigmoid->set_min_score(params[3]);
|
||||
}
|
||||
return absl::OkStatus();
|
||||
}
|
||||
} // namespace
|
||||
|
||||
absl::Status ConfigureScoreCalibration(
|
||||
tflite::ScoreTransformationType score_transformation, float default_score,
|
||||
absl::string_view score_calibration_file,
|
||||
ScoreCalibrationCalculatorOptions* calculator_options) {
|
||||
calculator_options->set_score_transformation(
|
||||
ConvertScoreTransformationType(score_transformation));
|
||||
calculator_options->set_default_score(default_score);
|
||||
|
||||
if (score_calibration_file.empty()) {
|
||||
return CreateStatusWithPayload(absl::StatusCode::kInvalidArgument,
|
||||
"Expected non-empty score calibration file.",
|
||||
MediaPipeTasksStatus::kInvalidArgumentError);
|
||||
}
|
||||
std::vector<absl::string_view> lines =
|
||||
absl::StrSplit(score_calibration_file, '\n');
|
||||
for (const auto& line : lines) {
|
||||
auto* sigmoid = calculator_options->add_sigmoids();
|
||||
MP_RETURN_IF_ERROR(FillSigmoidFromLine(line, sigmoid));
|
||||
}
|
||||
|
||||
return absl::OkStatus();
|
||||
}
|
||||
|
||||
} // namespace tasks
|
||||
} // namespace mediapipe
|
|
@ -0,0 +1,38 @@
|
|||
// Copyright 2022 The MediaPipe Authors.
|
||||
//
|
||||
// Licensed under the Apache License, Version 2.0 (the "License");
|
||||
// you may not use this file except in compliance with the License.
|
||||
// You may obtain a copy of the License at
|
||||
//
|
||||
// http://www.apache.org/licenses/LICENSE-2.0
|
||||
//
|
||||
// Unless required by applicable law or agreed to in writing, software
|
||||
// distributed under the License is distributed on an "AS IS" BASIS,
|
||||
// WITHOUT WARRANTIES OR CONDITIONS OF ANY KIND, either express or implied.
|
||||
// See the License for the specific language governing permissions and
|
||||
// limitations under the License.
|
||||
|
||||
#ifndef MEDIAPIPE_TASKS_CC_COMPONENTS_CALCULATORS_SCORE_CALIBRATION_UTILS_H_
|
||||
#define MEDIAPIPE_TASKS_CC_COMPONENTS_CALCULATORS_SCORE_CALIBRATION_UTILS_H_
|
||||
|
||||
#include "absl/status/status.h"
|
||||
#include "absl/strings/string_view.h"
|
||||
#include "mediapipe/tasks/cc/components/calculators/score_calibration_calculator.pb.h"
|
||||
#include "mediapipe/tasks/metadata/metadata_schema_generated.h"
|
||||
|
||||
namespace mediapipe {
|
||||
namespace tasks {
|
||||
|
||||
// Populates ScoreCalibrationCalculatorOptions given a TFLite Metadata score
|
||||
// transformation type, default threshold and score calibration AssociatedFile
|
||||
// contents, as specified in `TENSOR_AXIS_SCORE_CALIBRATION`:
|
||||
// https://github.com/google/mediapipe/blob/master/mediapipe/tasks/metadata/metadata_schema.fbs
|
||||
absl::Status ConfigureScoreCalibration(
|
||||
tflite::ScoreTransformationType score_transformation, float default_score,
|
||||
absl::string_view score_calibration_file,
|
||||
ScoreCalibrationCalculatorOptions* options);
|
||||
|
||||
} // namespace tasks
|
||||
} // namespace mediapipe
|
||||
|
||||
#endif // MEDIAPIPE_TASKS_CC_COMPONENTS_CALCULATORS_SCORE_CALIBRATION_UTILS_H_
|
|
@ -0,0 +1,130 @@
|
|||
// Copyright 2022 The MediaPipe Authors.
|
||||
//
|
||||
// Licensed under the Apache License, Version 2.0 (the "License");
|
||||
// you may not use this file except in compliance with the License.
|
||||
// You may obtain a copy of the License at
|
||||
//
|
||||
// http://www.apache.org/licenses/LICENSE-2.0
|
||||
//
|
||||
// Unless required by applicable law or agreed to in writing, software
|
||||
// distributed under the License is distributed on an "AS IS" BASIS,
|
||||
// WITHOUT WARRANTIES OR CONDITIONS OF ANY KIND, either express or implied.
|
||||
// See the License for the specific language governing permissions and
|
||||
// limitations under the License.
|
||||
|
||||
#include "mediapipe/tasks/cc/components/calculators/score_calibration_utils.h"
|
||||
|
||||
#include "absl/strings/str_cat.h"
|
||||
#include "mediapipe/framework/port/gmock.h"
|
||||
#include "mediapipe/framework/port/gtest.h"
|
||||
#include "mediapipe/framework/port/parse_text_proto.h"
|
||||
#include "mediapipe/framework/port/status_matchers.h"
|
||||
#include "mediapipe/tasks/cc/components/calculators/score_calibration_calculator.pb.h"
|
||||
#include "mediapipe/tasks/metadata/metadata_schema_generated.h"
|
||||
|
||||
namespace mediapipe {
|
||||
namespace tasks {
|
||||
namespace {
|
||||
|
||||
using ::testing::HasSubstr;
|
||||
|
||||
TEST(ConfigureScoreCalibrationTest, SucceedsWithoutTrailingNewline) {
|
||||
ScoreCalibrationCalculatorOptions options;
|
||||
std::string score_calibration_file =
|
||||
absl::StrCat("\n", "0.1,0.2,0.3\n", "0.4,0.5,0.6,0.7");
|
||||
|
||||
MP_ASSERT_OK(ConfigureScoreCalibration(
|
||||
tflite::ScoreTransformationType_IDENTITY,
|
||||
/*default_score=*/0.5, score_calibration_file, &options));
|
||||
|
||||
EXPECT_THAT(
|
||||
options,
|
||||
EqualsProto(ParseTextProtoOrDie<ScoreCalibrationCalculatorOptions>(R"pb(
|
||||
score_transformation: IDENTITY
|
||||
default_score: 0.5
|
||||
sigmoids {}
|
||||
sigmoids { scale: 0.1 slope: 0.2 offset: 0.3 }
|
||||
sigmoids { scale: 0.4 slope: 0.5 offset: 0.6 min_score: 0.7 }
|
||||
)pb")));
|
||||
}
|
||||
|
||||
TEST(ConfigureScoreCalibrationTest, SucceedsWithTrailingNewline) {
|
||||
ScoreCalibrationCalculatorOptions options;
|
||||
std::string score_calibration_file =
|
||||
absl::StrCat("\n", "0.1,0.2,0.3\n", "0.4,0.5,0.6,0.7\n");
|
||||
|
||||
MP_ASSERT_OK(ConfigureScoreCalibration(tflite::ScoreTransformationType_LOG,
|
||||
/*default_score=*/0.5,
|
||||
score_calibration_file, &options));
|
||||
|
||||
EXPECT_THAT(
|
||||
options,
|
||||
EqualsProto(ParseTextProtoOrDie<ScoreCalibrationCalculatorOptions>(R"pb(
|
||||
score_transformation: LOG
|
||||
default_score: 0.5
|
||||
sigmoids {}
|
||||
sigmoids { scale: 0.1 slope: 0.2 offset: 0.3 }
|
||||
sigmoids { scale: 0.4 slope: 0.5 offset: 0.6 min_score: 0.7 }
|
||||
sigmoids {}
|
||||
)pb")));
|
||||
}
|
||||
|
||||
TEST(ConfigureScoreCalibrationTest, FailsWithEmptyFile) {
|
||||
ScoreCalibrationCalculatorOptions options;
|
||||
|
||||
auto status =
|
||||
ConfigureScoreCalibration(tflite::ScoreTransformationType_LOG,
|
||||
/*default_score=*/0.5,
|
||||
/*score_calibration_file=*/"", &options);
|
||||
|
||||
EXPECT_EQ(status.code(), absl::StatusCode::kInvalidArgument);
|
||||
EXPECT_THAT(status.message(),
|
||||
HasSubstr("Expected non-empty score calibration file"));
|
||||
}
|
||||
|
||||
TEST(ConfigureScoreCalibrationTest, FailsWithInvalidNumParameters) {
|
||||
ScoreCalibrationCalculatorOptions options;
|
||||
std::string score_calibration_file = absl::StrCat("0.1,0.2,0.3\n", "0.1,0.2");
|
||||
|
||||
auto status = ConfigureScoreCalibration(tflite::ScoreTransformationType_LOG,
|
||||
/*default_score=*/0.5,
|
||||
score_calibration_file, &options);
|
||||
|
||||
EXPECT_EQ(status.code(), absl::StatusCode::kInvalidArgument);
|
||||
EXPECT_THAT(status.message(),
|
||||
HasSubstr("Expected 3 or 4 parameters per line"));
|
||||
}
|
||||
|
||||
TEST(ConfigureScoreCalibrationTest, FailsWithNonParseableParameter) {
|
||||
ScoreCalibrationCalculatorOptions options;
|
||||
std::string score_calibration_file =
|
||||
absl::StrCat("0.1,0.2,0.3\n", "0.1,foo,0.3\n");
|
||||
|
||||
auto status = ConfigureScoreCalibration(tflite::ScoreTransformationType_LOG,
|
||||
/*default_score=*/0.5,
|
||||
score_calibration_file, &options);
|
||||
|
||||
EXPECT_EQ(status.code(), absl::StatusCode::kInvalidArgument);
|
||||
EXPECT_THAT(
|
||||
status.message(),
|
||||
HasSubstr("Could not parse score calibration parameter as float"));
|
||||
}
|
||||
|
||||
TEST(ConfigureScoreCalibrationTest, FailsWithNegativeScaleParameter) {
|
||||
ScoreCalibrationCalculatorOptions options;
|
||||
std::string score_calibration_file =
|
||||
absl::StrCat("0.1,0.2,0.3\n", "-0.1,0.2,0.3\n");
|
||||
|
||||
auto status = ConfigureScoreCalibration(tflite::ScoreTransformationType_LOG,
|
||||
/*default_score=*/0.5,
|
||||
score_calibration_file, &options);
|
||||
|
||||
EXPECT_EQ(status.code(), absl::StatusCode::kInvalidArgument);
|
||||
EXPECT_THAT(
|
||||
status.message(),
|
||||
HasSubstr("The scale parameter of the sigmoids must be positive"));
|
||||
}
|
||||
|
||||
} // namespace
|
||||
} // namespace tasks
|
||||
} // namespace mediapipe
|
|
@ -159,12 +159,12 @@ absl::Status TensorsToSegmentationCalculator::Process(
|
|||
std::tie(output_width, output_height) = kOutputSizeIn(cc).Get();
|
||||
}
|
||||
Shape output_shape = {
|
||||
.height = output_height,
|
||||
.width = output_width,
|
||||
.channels = options_.segmenter_options().output_type() ==
|
||||
SegmenterOptions::CATEGORY_MASK
|
||||
? 1
|
||||
: input_shape.channels};
|
||||
/* height= */ output_height,
|
||||
/* width= */ output_width,
|
||||
/* channels= */ options_.segmenter_options().output_type() ==
|
||||
SegmenterOptions::CATEGORY_MASK
|
||||
? 1
|
||||
: input_shape.channels};
|
||||
|
||||
std::vector<Image> segmented_masks = GetSegmentationResult(
|
||||
input_shape, output_shape, input_tensor.GetCpuReadView().buffer<float>());
|
||||
|
|
|
@ -148,8 +148,9 @@ absl::StatusOr<ClassificationHeadsProperties> GetClassificationHeadsProperties(
|
|||
num_output_tensors, output_tensors_metadata->size()),
|
||||
MediaPipeTasksStatus::kMetadataInconsistencyError);
|
||||
}
|
||||
return ClassificationHeadsProperties{.num_heads = num_output_tensors,
|
||||
.quantized = num_quantized_tensors > 0};
|
||||
return ClassificationHeadsProperties{
|
||||
/* num_heads= */ num_output_tensors,
|
||||
/* quantized= */ num_quantized_tensors > 0};
|
||||
}
|
||||
|
||||
// Builds the label map from the tensor metadata, if available.
|
||||
|
|
|
@ -226,12 +226,14 @@ class ImagePreprocessingSubgraph : public Subgraph {
|
|||
|
||||
// Connect outputs.
|
||||
return {
|
||||
.tensors = image_to_tensor[Output<std::vector<Tensor>>(kTensorsTag)],
|
||||
.matrix = image_to_tensor[Output<std::array<float, 16>>(kMatrixTag)],
|
||||
.letterbox_padding =
|
||||
image_to_tensor[Output<std::array<float, 4>>(kLetterboxPaddingTag)],
|
||||
.image_size = image_size[Output<std::pair<int, int>>(kSizeTag)],
|
||||
.image = pass_through[Output<Image>("")],
|
||||
/* tensors= */ image_to_tensor[Output<std::vector<Tensor>>(
|
||||
kTensorsTag)],
|
||||
/* matrix= */
|
||||
image_to_tensor[Output<std::array<float, 16>>(kMatrixTag)],
|
||||
/* letterbox_padding= */
|
||||
image_to_tensor[Output<std::array<float, 4>>(kLetterboxPaddingTag)],
|
||||
/* image_size= */ image_size[Output<std::pair<int, int>>(kSizeTag)],
|
||||
/* image= */ pass_through[Output<Image>("")],
|
||||
};
|
||||
}
|
||||
};
|
||||
|
|
|
@ -18,8 +18,18 @@ limitations under the License.
|
|||
#include <errno.h>
|
||||
#include <fcntl.h>
|
||||
#include <stddef.h>
|
||||
|
||||
#ifdef ABSL_HAVE_MMAP
|
||||
#include <sys/mman.h>
|
||||
#endif
|
||||
|
||||
#ifdef _WIN32
|
||||
#include <direct.h>
|
||||
#include <io.h>
|
||||
#include <windows.h>
|
||||
#else
|
||||
#include <unistd.h>
|
||||
#endif
|
||||
|
||||
#include <memory>
|
||||
#include <string>
|
||||
|
@ -44,12 +54,17 @@ using ::absl::StatusCode;
|
|||
// file descriptor correctly, as according to mmap(2), the offset used in mmap
|
||||
// must be a multiple of sysconf(_SC_PAGE_SIZE).
|
||||
int64 GetPageSizeAlignedOffset(int64 offset) {
|
||||
#ifdef _WIN32
|
||||
// mmap is not used on Windows
|
||||
return -1;
|
||||
#else
|
||||
int64 aligned_offset = offset;
|
||||
int64 page_size = sysconf(_SC_PAGE_SIZE);
|
||||
if (offset % page_size != 0) {
|
||||
aligned_offset = offset / page_size * page_size;
|
||||
}
|
||||
return aligned_offset;
|
||||
#endif
|
||||
}
|
||||
|
||||
} // namespace
|
||||
|
@ -69,6 +84,12 @@ ExternalFileHandler::CreateFromExternalFile(
|
|||
}
|
||||
|
||||
absl::Status ExternalFileHandler::MapExternalFile() {
|
||||
// TODO: Add Windows support
|
||||
#ifdef _WIN32
|
||||
return CreateStatusWithPayload(StatusCode::kFailedPrecondition,
|
||||
"File loading is not yet supported on Windows",
|
||||
MediaPipeTasksStatus::kFileReadError);
|
||||
#else
|
||||
if (!external_file_.file_content().empty()) {
|
||||
return absl::OkStatus();
|
||||
}
|
||||
|
@ -169,6 +190,7 @@ absl::Status ExternalFileHandler::MapExternalFile() {
|
|||
MediaPipeTasksStatus::kFileMmapError);
|
||||
}
|
||||
return absl::OkStatus();
|
||||
#endif
|
||||
}
|
||||
|
||||
absl::string_view ExternalFileHandler::GetFileContent() {
|
||||
|
@ -182,9 +204,11 @@ absl::string_view ExternalFileHandler::GetFileContent() {
|
|||
}
|
||||
|
||||
ExternalFileHandler::~ExternalFileHandler() {
|
||||
#ifndef _WIN32
|
||||
if (buffer_ != MAP_FAILED) {
|
||||
munmap(buffer_, buffer_aligned_size_);
|
||||
}
|
||||
#endif
|
||||
if (owned_fd_ >= 0) {
|
||||
close(owned_fd_);
|
||||
}
|
||||
|
|
|
@ -12,7 +12,7 @@ WITHOUT WARRANTIES OR CONDITIONS OF ANY KIND, either express or implied.
|
|||
See the License for the specific language governing permissions and
|
||||
limitations under the License.
|
||||
==============================================================================*/
|
||||
|
||||
// TODO Refactor naming and class structure of hand related Tasks.
|
||||
syntax = "proto2";
|
||||
|
||||
package mediapipe.tasks.vision.hand_gesture_recognizer.proto;
|
||||
|
|
|
@ -388,13 +388,13 @@ class HandLandmarkDetectorGraph : public core::ModelTaskGraph {
|
|||
hand_rect_transformation[Output<NormalizedRect>("")];
|
||||
|
||||
return {{
|
||||
.hand_landmarks = projected_landmarks,
|
||||
.world_hand_landmarks = projected_world_landmarks,
|
||||
.hand_rect_next_frame = hand_rect_next_frame,
|
||||
.hand_presence = hand_presence,
|
||||
.hand_presence_score = hand_presence_score,
|
||||
.handedness = handedness,
|
||||
.image_size = image_size,
|
||||
/* hand_landmarks= */ projected_landmarks,
|
||||
/* world_hand_landmarks= */ projected_world_landmarks,
|
||||
/* hand_rect_next_frame= */ hand_rect_next_frame,
|
||||
/* hand_presence= */ hand_presence,
|
||||
/* hand_presence_score= */ hand_presence_score,
|
||||
/* handedness= */ handedness,
|
||||
/* image_size= */ image_size,
|
||||
}};
|
||||
}
|
||||
};
|
||||
|
|
|
@ -24,7 +24,7 @@ message HandLandmarkDetectorOptions {
|
|||
extend mediapipe.CalculatorOptions {
|
||||
optional HandLandmarkDetectorOptions ext = 462713202;
|
||||
}
|
||||
// Base options for configuring Task library, such as specifying the TfLite
|
||||
// Base options for configuring MediaPipe Tasks, such as specifying the TfLite
|
||||
// model file with metadata, accelerator options, etc.
|
||||
optional core.proto.BaseOptions base_options = 1;
|
||||
|
||||
|
|
|
@ -25,7 +25,7 @@ message ImageClassifierOptions {
|
|||
extend mediapipe.CalculatorOptions {
|
||||
optional ImageClassifierOptions ext = 456383383;
|
||||
}
|
||||
// Base options for configuring Task library, such as specifying the TfLite
|
||||
// Base options for configuring MediaPipe Tasks, such as specifying the TfLite
|
||||
// model file with metadata, accelerator options, etc.
|
||||
optional core.proto.BaseOptions base_options = 1;
|
||||
|
||||
|
|
|
@ -142,7 +142,7 @@ TEST_F(CreateTest, FailsWithSelectiveOpResolverMissingOps) {
|
|||
// interpreter errors (e.g., "Encountered unresolved custom op").
|
||||
EXPECT_EQ(image_classifier_or.status().code(), absl::StatusCode::kInternal);
|
||||
EXPECT_THAT(image_classifier_or.status().message(),
|
||||
HasSubstr("interpreter_builder(&interpreter_) == kTfLiteOk"));
|
||||
HasSubstr("interpreter_builder(&interpreter) == kTfLiteOk"));
|
||||
}
|
||||
TEST_F(CreateTest, FailsWithMissingModel) {
|
||||
auto image_classifier_or =
|
||||
|
|
|
@ -12,34 +12,25 @@
|
|||
# See the License for the specific language governing permissions and
|
||||
# limitations under the License.
|
||||
|
||||
load("//mediapipe/framework/port:build_config.bzl", "mediapipe_proto_library")
|
||||
|
||||
package(default_visibility = ["//mediapipe/tasks:internal"])
|
||||
|
||||
licenses(["notice"])
|
||||
|
||||
mediapipe_proto_library(
|
||||
name = "image_segmenter_options_proto",
|
||||
srcs = ["image_segmenter_options.proto"],
|
||||
deps = [
|
||||
"//mediapipe/framework:calculator_options_proto",
|
||||
"//mediapipe/framework:calculator_proto",
|
||||
"//mediapipe/tasks/cc/components:segmenter_options_proto",
|
||||
"//mediapipe/tasks/cc/core/proto:base_options_proto",
|
||||
],
|
||||
)
|
||||
|
||||
cc_library(
|
||||
name = "image_segmenter",
|
||||
srcs = ["image_segmenter.cc"],
|
||||
hdrs = ["image_segmenter.h"],
|
||||
deps = [
|
||||
":image_segmenter_graph",
|
||||
":image_segmenter_options_cc_proto",
|
||||
"//mediapipe/framework/api2:builder",
|
||||
"//mediapipe/framework/formats:image",
|
||||
"//mediapipe/tasks/cc/core:base_task_api",
|
||||
"//mediapipe/tasks/cc/core:task_api_factory",
|
||||
"//mediapipe/tasks/cc/components:segmenter_options_cc_proto",
|
||||
"//mediapipe/tasks/cc/core:base_options",
|
||||
"//mediapipe/tasks/cc/core:utils",
|
||||
"//mediapipe/tasks/cc/vision/core:base_vision_task_api",
|
||||
"//mediapipe/tasks/cc/vision/core:running_mode",
|
||||
"//mediapipe/tasks/cc/vision/core:vision_task_api_factory",
|
||||
"//mediapipe/tasks/cc/vision/image_segmenter/proto:image_segmenter_options_cc_proto",
|
||||
"@com_google_absl//absl/memory",
|
||||
"@com_google_absl//absl/status:statusor",
|
||||
"@org_tensorflow//tensorflow/lite/core/api:op_resolver",
|
||||
|
@ -51,7 +42,6 @@ cc_library(
|
|||
name = "image_segmenter_graph",
|
||||
srcs = ["image_segmenter_graph.cc"],
|
||||
deps = [
|
||||
":image_segmenter_options_cc_proto",
|
||||
"//mediapipe/calculators/core:merge_to_vector_calculator",
|
||||
"//mediapipe/calculators/image:image_properties_calculator",
|
||||
"//mediapipe/calculators/tensor:image_to_tensor_calculator",
|
||||
|
@ -70,6 +60,7 @@ cc_library(
|
|||
"//mediapipe/tasks/cc/core:model_task_graph",
|
||||
"//mediapipe/tasks/cc/core/proto:inference_subgraph_cc_proto",
|
||||
"//mediapipe/tasks/cc/metadata:metadata_extractor",
|
||||
"//mediapipe/tasks/cc/vision/image_segmenter/proto:image_segmenter_options_cc_proto",
|
||||
"//mediapipe/tasks/metadata:metadata_schema_cc",
|
||||
"//mediapipe/util:label_map_cc_proto",
|
||||
"//mediapipe/util:label_map_util",
|
||||
|
@ -82,9 +73,9 @@ cc_library(
|
|||
)
|
||||
|
||||
cc_library(
|
||||
name = "custom_op_resolvers",
|
||||
srcs = ["custom_op_resolvers.cc"],
|
||||
hdrs = ["custom_op_resolvers.h"],
|
||||
name = "image_segmenter_op_resolvers",
|
||||
srcs = ["image_segmenter_op_resolvers.cc"],
|
||||
hdrs = ["image_segmenter_op_resolvers.h"],
|
||||
deps = [
|
||||
"//mediapipe/util/tflite/operations:landmarks_to_transform_matrix",
|
||||
"//mediapipe/util/tflite/operations:max_pool_argmax",
|
134
mediapipe/tasks/cc/vision/image_segmenter/image_segmenter.cc
Normal file
134
mediapipe/tasks/cc/vision/image_segmenter/image_segmenter.cc
Normal file
|
@ -0,0 +1,134 @@
|
|||
/* Copyright 2022 The MediaPipe Authors. All Rights Reserved.
|
||||
|
||||
Licensed under the Apache License, Version 2.0 (the "License");
|
||||
you may not use this file except in compliance with the License.
|
||||
You may obtain a copy of the License at
|
||||
|
||||
http://www.apache.org/licenses/LICENSE-2.0
|
||||
|
||||
Unless required by applicable law or agreed to in writing, software
|
||||
distributed under the License is distributed on an "AS IS" BASIS,
|
||||
WITHOUT WARRANTIES OR CONDITIONS OF ANY KIND, either express or implied.
|
||||
See the License for the specific language governing permissions and
|
||||
limitations under the License.
|
||||
==============================================================================*/
|
||||
|
||||
#include "mediapipe/tasks/cc/vision/image_segmenter/image_segmenter.h"
|
||||
|
||||
#include "mediapipe/framework/api2/builder.h"
|
||||
#include "mediapipe/framework/formats/image.h"
|
||||
#include "mediapipe/tasks/cc/components/segmenter_options.pb.h"
|
||||
#include "mediapipe/tasks/cc/core/utils.h"
|
||||
#include "mediapipe/tasks/cc/vision/core/running_mode.h"
|
||||
#include "mediapipe/tasks/cc/vision/core/vision_task_api_factory.h"
|
||||
|
||||
namespace mediapipe {
|
||||
namespace tasks {
|
||||
namespace vision {
|
||||
namespace {
|
||||
|
||||
constexpr char kSegmentationStreamName[] = "segmented_mask_out";
|
||||
constexpr char kGroupedSegmentationTag[] = "GROUPED_SEGMENTATION";
|
||||
constexpr char kImageInStreamName[] = "image_in";
|
||||
constexpr char kImageOutStreamName[] = "image_out";
|
||||
constexpr char kImageTag[] = "IMAGE";
|
||||
constexpr char kSubgraphTypeName[] =
|
||||
"mediapipe.tasks.vision.ImageSegmenterGraph";
|
||||
|
||||
using ::mediapipe::CalculatorGraphConfig;
|
||||
using ::mediapipe::Image;
|
||||
using ImageSegmenterOptionsProto =
|
||||
image_segmenter::proto::ImageSegmenterOptions;
|
||||
|
||||
// Creates a MediaPipe graph config that only contains a single subgraph node of
|
||||
// "mediapipe.tasks.vision.ImageSegmenterGraph".
|
||||
CalculatorGraphConfig CreateGraphConfig(
|
||||
std::unique_ptr<ImageSegmenterOptionsProto> options,
|
||||
bool enable_flow_limiting) {
|
||||
api2::builder::Graph graph;
|
||||
auto& task_subgraph = graph.AddNode(kSubgraphTypeName);
|
||||
task_subgraph.GetOptions<ImageSegmenterOptionsProto>().Swap(options.get());
|
||||
graph.In(kImageTag).SetName(kImageInStreamName);
|
||||
task_subgraph.Out(kGroupedSegmentationTag).SetName(kSegmentationStreamName) >>
|
||||
graph.Out(kGroupedSegmentationTag);
|
||||
task_subgraph.Out(kImageTag).SetName(kImageOutStreamName) >>
|
||||
graph.Out(kImageTag);
|
||||
if (enable_flow_limiting) {
|
||||
return tasks::core::AddFlowLimiterCalculator(
|
||||
graph, task_subgraph, {kImageTag}, kGroupedSegmentationTag);
|
||||
}
|
||||
graph.In(kImageTag) >> task_subgraph.In(kImageTag);
|
||||
return graph.GetConfig();
|
||||
}
|
||||
|
||||
// Converts the user-facing ImageSegmenterOptions struct to the internal
|
||||
// ImageSegmenterOptions proto.
|
||||
std::unique_ptr<ImageSegmenterOptionsProto> ConvertImageSegmenterOptionsToProto(
|
||||
ImageSegmenterOptions* options) {
|
||||
auto options_proto = std::make_unique<ImageSegmenterOptionsProto>();
|
||||
auto base_options_proto = std::make_unique<tasks::core::proto::BaseOptions>(
|
||||
tasks::core::ConvertBaseOptionsToProto(&(options->base_options)));
|
||||
options_proto->mutable_base_options()->Swap(base_options_proto.get());
|
||||
options_proto->mutable_base_options()->set_use_stream_mode(
|
||||
options->running_mode != core::RunningMode::IMAGE);
|
||||
options_proto->set_display_names_locale(options->display_names_locale);
|
||||
switch (options->output_type) {
|
||||
case ImageSegmenterOptions::OutputType::CATEGORY_MASK:
|
||||
options_proto->mutable_segmenter_options()->set_output_type(
|
||||
SegmenterOptions::CATEGORY_MASK);
|
||||
break;
|
||||
case ImageSegmenterOptions::OutputType::CONFIDENCE_MASK:
|
||||
options_proto->mutable_segmenter_options()->set_output_type(
|
||||
SegmenterOptions::CONFIDENCE_MASK);
|
||||
break;
|
||||
}
|
||||
switch (options->activation) {
|
||||
case ImageSegmenterOptions::Activation::NONE:
|
||||
options_proto->mutable_segmenter_options()->set_activation(
|
||||
SegmenterOptions::NONE);
|
||||
break;
|
||||
case ImageSegmenterOptions::Activation::SIGMOID:
|
||||
options_proto->mutable_segmenter_options()->set_activation(
|
||||
SegmenterOptions::SIGMOID);
|
||||
break;
|
||||
case ImageSegmenterOptions::Activation::SOFTMAX:
|
||||
options_proto->mutable_segmenter_options()->set_activation(
|
||||
SegmenterOptions::SOFTMAX);
|
||||
break;
|
||||
}
|
||||
return options_proto;
|
||||
}
|
||||
|
||||
} // namespace
|
||||
|
||||
absl::StatusOr<std::unique_ptr<ImageSegmenter>> ImageSegmenter::Create(
|
||||
std::unique_ptr<ImageSegmenterOptions> options) {
|
||||
auto options_proto = ConvertImageSegmenterOptionsToProto(options.get());
|
||||
tasks::core::PacketsCallback packets_callback = nullptr;
|
||||
return core::VisionTaskApiFactory::Create<ImageSegmenter,
|
||||
ImageSegmenterOptionsProto>(
|
||||
CreateGraphConfig(
|
||||
std::move(options_proto),
|
||||
options->running_mode == core::RunningMode::LIVE_STREAM),
|
||||
std::move(options->base_options.op_resolver), options->running_mode,
|
||||
std::move(packets_callback));
|
||||
}
|
||||
|
||||
absl::StatusOr<std::vector<Image>> ImageSegmenter::Segment(
|
||||
mediapipe::Image image) {
|
||||
if (image.UsesGpu()) {
|
||||
return CreateStatusWithPayload(
|
||||
absl::StatusCode::kInvalidArgument,
|
||||
absl::StrCat("GPU input images are currently not supported."),
|
||||
MediaPipeTasksStatus::kRunnerUnexpectedInputError);
|
||||
}
|
||||
ASSIGN_OR_RETURN(
|
||||
auto output_packets,
|
||||
ProcessImageData({{kImageInStreamName,
|
||||
mediapipe::MakePacket<Image>(std::move(image))}}));
|
||||
return output_packets[kSegmentationStreamName].Get<std::vector<Image>>();
|
||||
}
|
||||
|
||||
} // namespace vision
|
||||
} // namespace tasks
|
||||
} // namespace mediapipe
|
123
mediapipe/tasks/cc/vision/image_segmenter/image_segmenter.h
Normal file
123
mediapipe/tasks/cc/vision/image_segmenter/image_segmenter.h
Normal file
|
@ -0,0 +1,123 @@
|
|||
/* Copyright 2022 The MediaPipe Authors. All Rights Reserved.
|
||||
|
||||
Licensed under the Apache License, Version 2.0 (the "License");
|
||||
you may not use this file except in compliance with the License.
|
||||
You may obtain a copy of the License at
|
||||
|
||||
http://www.apache.org/licenses/LICENSE-2.0
|
||||
|
||||
Unless required by applicable law or agreed to in writing, software
|
||||
distributed under the License is distributed on an "AS IS" BASIS,
|
||||
WITHOUT WARRANTIES OR CONDITIONS OF ANY KIND, either express or implied.
|
||||
See the License for the specific language governing permissions and
|
||||
limitations under the License.
|
||||
==============================================================================*/
|
||||
|
||||
#ifndef MEDIAPIPE_TASKS_CC_VISION_IMAGE_SEGMENTER_IMAGE_SEGMENTER_H_
|
||||
#define MEDIAPIPE_TASKS_CC_VISION_IMAGE_SEGMENTER_IMAGE_SEGMENTER_H_
|
||||
|
||||
#include <memory>
|
||||
#include <utility>
|
||||
#include <vector>
|
||||
|
||||
#include "absl/memory/memory.h"
|
||||
#include "absl/status/statusor.h"
|
||||
#include "mediapipe/framework/formats/image.h"
|
||||
#include "mediapipe/tasks/cc/core/base_options.h"
|
||||
#include "mediapipe/tasks/cc/vision/core/base_vision_task_api.h"
|
||||
#include "mediapipe/tasks/cc/vision/image_segmenter/proto/image_segmenter_options.pb.h"
|
||||
#include "tensorflow/lite/core/api/op_resolver.h"
|
||||
#include "tensorflow/lite/kernels/register.h"
|
||||
|
||||
namespace mediapipe {
|
||||
namespace tasks {
|
||||
namespace vision {
|
||||
|
||||
// The options for configuring a mediapipe image segmenter task.
|
||||
struct ImageSegmenterOptions {
|
||||
// Base options for configuring MediaPipe Tasks, such as specifying the model
|
||||
// file with metadata, accelerator options, op resolver, etc.
|
||||
tasks::core::BaseOptions base_options;
|
||||
|
||||
// The running mode of the task. Default to the image mode.
|
||||
// Image segmenter has three running modes:
|
||||
// 1) The image mode for segmenting image on single image inputs.
|
||||
// 2) The video mode for segmenting image on the decoded frames of a video.
|
||||
// 3) The live stream mode for segmenting image on the live stream of input
|
||||
// data, such as from camera. In this mode, the "result_callback" below must
|
||||
// be specified to receive the segmentation results asynchronously.
|
||||
core::RunningMode running_mode = core::RunningMode::IMAGE;
|
||||
|
||||
// The locale to use for display names specified through the TFLite Model
|
||||
// Metadata, if any. Defaults to English.
|
||||
std::string display_names_locale = "en";
|
||||
|
||||
// The output type of segmentation results.
|
||||
enum OutputType {
|
||||
// Gives a single output mask where each pixel represents the class which
|
||||
// the pixel in the original image was predicted to belong to.
|
||||
CATEGORY_MASK = 0,
|
||||
// Gives a list of output masks where, for each mask, each pixel represents
|
||||
// the prediction confidence, usually in the [0, 1] range.
|
||||
CONFIDENCE_MASK = 1,
|
||||
};
|
||||
|
||||
OutputType output_type = OutputType::CATEGORY_MASK;
|
||||
|
||||
// The activation function used on the raw segmentation model output.
|
||||
enum Activation {
|
||||
NONE = 0, // No activation function is used.
|
||||
SIGMOID = 1, // Assumes 1-channel input tensor.
|
||||
SOFTMAX = 2, // Assumes multi-channel input tensor.
|
||||
};
|
||||
|
||||
Activation activation = Activation::NONE;
|
||||
|
||||
// The user-defined result callback for processing live stream data.
|
||||
// The result callback should only be specified when the running mode is set
|
||||
// to RunningMode::LIVE_STREAM.
|
||||
std::function<void(absl::StatusOr<std::vector<mediapipe::Image>>,
|
||||
const Image&, int64)>
|
||||
result_callback = nullptr;
|
||||
};
|
||||
|
||||
// Performs segmentation on images.
|
||||
//
|
||||
// The API expects a TFLite model with mandatory TFLite Model Metadata.
|
||||
//
|
||||
// Input tensor:
|
||||
// (kTfLiteUInt8/kTfLiteFloat32)
|
||||
// - image input of size `[batch x height x width x channels]`.
|
||||
// - batch inference is not supported (`batch` is required to be 1).
|
||||
// - RGB and greyscale inputs are supported (`channels` is required to be
|
||||
// 1 or 3).
|
||||
// - if type is kTfLiteFloat32, NormalizationOptions are required to be
|
||||
// attached to the metadata for input normalization.
|
||||
// Output tensors:
|
||||
// (kTfLiteUInt8/kTfLiteFloat32)
|
||||
// - list of segmented masks.
|
||||
// - if `output_type` is CATEGORY_MASK, uint8 Image, Image vector of size 1.
|
||||
// - if `output_type` is CONFIDENCE_MASK, float32 Image list of size
|
||||
// `cahnnels`.
|
||||
// - batch is always 1
|
||||
// An example of such model can be found at:
|
||||
// https://tfhub.dev/tensorflow/lite-model/deeplabv3/1/metadata/2
|
||||
class ImageSegmenter : tasks::vision::core::BaseVisionTaskApi {
|
||||
public:
|
||||
using BaseVisionTaskApi::BaseVisionTaskApi;
|
||||
|
||||
// Creates an ImageSegmenter from the provided options. A non-default
|
||||
// OpResolver can be specified in the BaseOptions of ImageSegmenterOptions,
|
||||
// to support custom Ops of the segmentation model.
|
||||
static absl::StatusOr<std::unique_ptr<ImageSegmenter>> Create(
|
||||
std::unique_ptr<ImageSegmenterOptions> options);
|
||||
|
||||
// Runs the actual segmentation task.
|
||||
absl::StatusOr<std::vector<mediapipe::Image>> Segment(mediapipe::Image image);
|
||||
};
|
||||
|
||||
} // namespace vision
|
||||
} // namespace tasks
|
||||
} // namespace mediapipe
|
||||
|
||||
#endif // MEDIAPIPE_TASKS_CC_VISION_IMAGE_SEGMENTER_IMAGE_SEGMENTER_H_
|
|
@ -33,7 +33,7 @@ limitations under the License.
|
|||
#include "mediapipe/tasks/cc/core/model_task_graph.h"
|
||||
#include "mediapipe/tasks/cc/core/proto/inference_subgraph.pb.h"
|
||||
#include "mediapipe/tasks/cc/metadata/metadata_extractor.h"
|
||||
#include "mediapipe/tasks/cc/vision/segmentation/image_segmenter_options.pb.h"
|
||||
#include "mediapipe/tasks/cc/vision/image_segmenter/proto/image_segmenter_options.pb.h"
|
||||
#include "mediapipe/tasks/metadata/metadata_schema_generated.h"
|
||||
#include "mediapipe/util/label_map.pb.h"
|
||||
#include "mediapipe/util/label_map_util.h"
|
||||
|
@ -53,6 +53,7 @@ using ::mediapipe::api2::builder::MultiSource;
|
|||
using ::mediapipe::api2::builder::Source;
|
||||
using ::mediapipe::tasks::SegmenterOptions;
|
||||
using ::mediapipe::tasks::metadata::ModelMetadataExtractor;
|
||||
using ::mediapipe::tasks::vision::image_segmenter::proto::ImageSegmenterOptions;
|
||||
using ::tflite::Tensor;
|
||||
using ::tflite::TensorMetadata;
|
||||
using LabelItems = mediapipe::proto_ns::Map<int64, ::mediapipe::LabelMapItem>;
|
||||
|
@ -63,6 +64,14 @@ constexpr char kImageTag[] = "IMAGE";
|
|||
constexpr char kTensorsTag[] = "TENSORS";
|
||||
constexpr char kOutputSizeTag[] = "OUTPUT_SIZE";
|
||||
|
||||
// Struct holding the different output streams produced by the image segmenter
|
||||
// subgraph.
|
||||
struct ImageSegmenterOutputs {
|
||||
std::vector<Source<Image>> segmented_masks;
|
||||
// The same as the input image, mainly used for live stream mode.
|
||||
Source<Image> image;
|
||||
};
|
||||
|
||||
} // namespace
|
||||
|
||||
absl::Status SanityCheckOptions(const ImageSegmenterOptions& options) {
|
||||
|
@ -140,6 +149,10 @@ absl::StatusOr<const Tensor*> GetOutputTensor(
|
|||
|
||||
// An "mediapipe.tasks.vision.ImageSegmenterGraph" performs semantic
|
||||
// segmentation.
|
||||
// Two kinds of outputs are provided: SEGMENTATION and GROUPED_SEGMENTATION.
|
||||
// Users can retrieve segmented mask of only particular category/channel from
|
||||
// SEGMENTATION, and users can also get all segmented masks from
|
||||
// GROUPED_SEGMENTATION.
|
||||
// - Accepts CPU input images and outputs segmented masks on CPU.
|
||||
//
|
||||
// Inputs:
|
||||
|
@ -147,8 +160,13 @@ absl::StatusOr<const Tensor*> GetOutputTensor(
|
|||
// Image to perform segmentation on.
|
||||
//
|
||||
// Outputs:
|
||||
// SEGMENTATION - SEGMENTATION
|
||||
// Segmented masks.
|
||||
// SEGMENTATION - mediapipe::Image @Multiple
|
||||
// Segmented masks for individual category. Segmented mask of single
|
||||
// category can be accessed by index based output stream.
|
||||
// GROUPED_SEGMENTATION - std::vector<mediapipe::Image>
|
||||
// The output segmented masks grouped in a vector.
|
||||
// IMAGE - mediapipe::Image
|
||||
// The image that image segmenter runs on.
|
||||
//
|
||||
// Example:
|
||||
// node {
|
||||
|
@ -156,7 +174,8 @@ absl::StatusOr<const Tensor*> GetOutputTensor(
|
|||
// input_stream: "IMAGE:image"
|
||||
// output_stream: "SEGMENTATION:segmented_masks"
|
||||
// options {
|
||||
// [mediapipe.tasks.ImageSegmenterOptions.ext] {
|
||||
// [mediapipe.tasks.vision.image_segmenter.proto.ImageSegmenterOptions.ext]
|
||||
// {
|
||||
// segmenter_options {
|
||||
// output_type: CONFIDENCE_MASK
|
||||
// activation: SOFTMAX
|
||||
|
@ -171,20 +190,22 @@ class ImageSegmenterGraph : public core::ModelTaskGraph {
|
|||
ASSIGN_OR_RETURN(const auto* model_resources,
|
||||
CreateModelResources<ImageSegmenterOptions>(sc));
|
||||
Graph graph;
|
||||
ASSIGN_OR_RETURN(auto segmentations,
|
||||
ASSIGN_OR_RETURN(auto output_streams,
|
||||
BuildSegmentationTask(
|
||||
sc->Options<ImageSegmenterOptions>(), *model_resources,
|
||||
graph[Input<Image>(kImageTag)], graph));
|
||||
|
||||
auto& merge_images_to_vector =
|
||||
graph.AddNode("MergeImagesToVectorCalculator");
|
||||
for (int i = 0; i < segmentations.size(); ++i) {
|
||||
segmentations[i] >> merge_images_to_vector[Input<Image>::Multiple("")][i];
|
||||
segmentations[i] >> graph[Output<Image>::Multiple(kSegmentationTag)][i];
|
||||
for (int i = 0; i < output_streams.segmented_masks.size(); ++i) {
|
||||
output_streams.segmented_masks[i] >>
|
||||
merge_images_to_vector[Input<Image>::Multiple("")][i];
|
||||
output_streams.segmented_masks[i] >>
|
||||
graph[Output<Image>::Multiple(kSegmentationTag)][i];
|
||||
}
|
||||
merge_images_to_vector.Out("") >>
|
||||
graph[Output<std::vector<Image>>(kGroupedSegmentationTag)];
|
||||
|
||||
output_streams.image >> graph[Output<Image>(kImageTag)];
|
||||
return graph.GetConfig();
|
||||
}
|
||||
|
||||
|
@ -193,12 +214,12 @@ class ImageSegmenterGraph : public core::ModelTaskGraph {
|
|||
// builder::Graph instance. The segmentation pipeline takes images
|
||||
// (mediapipe::Image) as the input and returns segmented image mask as output.
|
||||
//
|
||||
// task_options: the mediapipe tasks ImageSegmenterOptions.
|
||||
// task_options: the mediapipe tasks ImageSegmenterOptions proto.
|
||||
// model_resources: the ModelSources object initialized from a segmentation
|
||||
// model file with model metadata.
|
||||
// image_in: (mediapipe::Image) stream to run segmentation on.
|
||||
// graph: the mediapipe builder::Graph instance to be updated.
|
||||
absl::StatusOr<std::vector<Source<Image>>> BuildSegmentationTask(
|
||||
absl::StatusOr<ImageSegmenterOutputs> BuildSegmentationTask(
|
||||
const ImageSegmenterOptions& task_options,
|
||||
const core::ModelResources& model_resources, Source<Image> image_in,
|
||||
Graph& graph) {
|
||||
|
@ -246,7 +267,10 @@ class ImageSegmenterGraph : public core::ModelTaskGraph {
|
|||
tensor_to_images[Output<Image>::Multiple(kSegmentationTag)][i]));
|
||||
}
|
||||
}
|
||||
return segmented_masks;
|
||||
return {{
|
||||
.segmented_masks = segmented_masks,
|
||||
.image = preprocessing[Output<Image>(kImageTag)],
|
||||
}};
|
||||
}
|
||||
};
|
||||
|
|
@ -13,7 +13,7 @@ See the License for the specific language governing permissions and
|
|||
limitations under the License.
|
||||
==============================================================================*/
|
||||
|
||||
#include "mediapipe/tasks/cc/vision/segmentation/custom_op_resolvers.h"
|
||||
#include "mediapipe/tasks/cc/vision/image_segmenter/image_segmenter_op_resolvers.h"
|
||||
|
||||
#include "mediapipe/util/tflite/operations/landmarks_to_transform_matrix.h"
|
||||
#include "mediapipe/util/tflite/operations/max_pool_argmax.h"
|
|
@ -13,8 +13,8 @@ See the License for the specific language governing permissions and
|
|||
limitations under the License.
|
||||
==============================================================================*/
|
||||
|
||||
#ifndef MEDIAPIPE_TASKS_CC_VISION_SEGMENTATION_CUSTOM_OP_RESOLVERS_H_
|
||||
#define MEDIAPIPE_TASKS_CC_VISION_SEGMENTATION_CUSTOM_OP_RESOLVERS_H_
|
||||
#ifndef MEDIAPIPE_TASKS_CC_VISION_IMAGE_SEGMENTER_IMAGE_SEGMENTER_OP_RESOLVERS_H_
|
||||
#define MEDIAPIPE_TASKS_CC_VISION_IMAGE_SEGMENTER_IMAGE_SEGMENTER_OP_RESOLVERS_H_
|
||||
|
||||
#include "tensorflow/lite/kernels/register.h"
|
||||
|
||||
|
@ -34,4 +34,4 @@ class SelfieSegmentationModelOpResolver
|
|||
} // namespace tasks
|
||||
} // namespace mediapipe
|
||||
|
||||
#endif // MEDIAPIPE_TASKS_CC_VISION_SEGMENTATION_CUSTOM_OP_RESOLVERS_H_
|
||||
#endif // MEDIAPIPE_TASKS_CC_VISION_IMAGE_SEGMENTER_IMAGE_SEGMENTER_OP_RESOLVERS_H_
|
|
@ -13,7 +13,7 @@ See the License for the specific language governing permissions and
|
|||
limitations under the License.
|
||||
==============================================================================*/
|
||||
|
||||
#include "mediapipe/tasks/cc/vision/segmentation/image_segmenter.h"
|
||||
#include "mediapipe/tasks/cc/vision/image_segmenter/image_segmenter.h"
|
||||
|
||||
#include <cstdint>
|
||||
#include <memory>
|
||||
|
@ -32,8 +32,8 @@ limitations under the License.
|
|||
#include "mediapipe/tasks/cc/components/segmenter_options.pb.h"
|
||||
#include "mediapipe/tasks/cc/core/proto/base_options.pb.h"
|
||||
#include "mediapipe/tasks/cc/core/proto/external_file.pb.h"
|
||||
#include "mediapipe/tasks/cc/vision/segmentation/custom_op_resolvers.h"
|
||||
#include "mediapipe/tasks/cc/vision/segmentation/image_segmenter_options.pb.h"
|
||||
#include "mediapipe/tasks/cc/vision/image_segmenter/image_segmenter_op_resolvers.h"
|
||||
#include "mediapipe/tasks/cc/vision/image_segmenter/proto/image_segmenter_options.pb.h"
|
||||
#include "mediapipe/tasks/cc/vision/utils/image_utils.h"
|
||||
#include "tensorflow/lite/core/shims/cc/shims_test_util.h"
|
||||
#include "tensorflow/lite/kernels/builtin_op_kernels.h"
|
||||
|
@ -46,11 +46,8 @@ namespace {
|
|||
|
||||
using ::mediapipe::Image;
|
||||
using ::mediapipe::file::JoinPath;
|
||||
using ::mediapipe::tasks::ImageSegmenterOptions;
|
||||
using ::mediapipe::tasks::SegmenterOptions;
|
||||
using ::testing::HasSubstr;
|
||||
using ::testing::Optional;
|
||||
using ::tflite::ops::builtin::BuiltinOpResolver;
|
||||
|
||||
constexpr char kTestDataDirectory[] = "/mediapipe/tasks/testdata/vision/";
|
||||
constexpr char kDeeplabV3WithMetadata[] = "deeplabv3.tflite";
|
||||
|
@ -167,25 +164,25 @@ class DeepLabOpResolverMissingOps : public ::tflite::MutableOpResolver {
|
|||
|
||||
TEST_F(CreateFromOptionsTest, SucceedsWithSelectiveOpResolver) {
|
||||
auto options = std::make_unique<ImageSegmenterOptions>();
|
||||
options->mutable_base_options()->mutable_model_file()->set_file_name(
|
||||
JoinPath("./", kTestDataDirectory, kDeeplabV3WithMetadata));
|
||||
MP_ASSERT_OK(ImageSegmenter::Create(std::move(options),
|
||||
absl::make_unique<DeepLabOpResolver>()));
|
||||
options->base_options.model_file_name =
|
||||
JoinPath("./", kTestDataDirectory, kDeeplabV3WithMetadata);
|
||||
options->base_options.op_resolver = absl::make_unique<DeepLabOpResolver>();
|
||||
MP_ASSERT_OK(ImageSegmenter::Create(std::move(options)));
|
||||
}
|
||||
|
||||
TEST_F(CreateFromOptionsTest, FailsWithSelectiveOpResolverMissingOps) {
|
||||
auto options = std::make_unique<ImageSegmenterOptions>();
|
||||
options->mutable_base_options()->mutable_model_file()->set_file_name(
|
||||
JoinPath("./", kTestDataDirectory, kDeeplabV3WithMetadata));
|
||||
|
||||
auto segmenter_or = ImageSegmenter::Create(
|
||||
std::move(options), absl::make_unique<DeepLabOpResolverMissingOps>());
|
||||
options->base_options.model_file_name =
|
||||
JoinPath("./", kTestDataDirectory, kDeeplabV3WithMetadata);
|
||||
options->base_options.op_resolver =
|
||||
absl::make_unique<DeepLabOpResolverMissingOps>();
|
||||
auto segmenter_or = ImageSegmenter::Create(std::move(options));
|
||||
// TODO: Make MediaPipe InferenceCalculator report the detailed
|
||||
// interpreter errors (e.g., "Encountered unresolved custom op").
|
||||
EXPECT_EQ(segmenter_or.status().code(), absl::StatusCode::kInternal);
|
||||
EXPECT_THAT(
|
||||
segmenter_or.status().message(),
|
||||
testing::HasSubstr("interpreter_builder(&interpreter_) == kTfLiteOk"));
|
||||
testing::HasSubstr("interpreter_builder(&interpreter) == kTfLiteOk"));
|
||||
}
|
||||
|
||||
TEST_F(CreateFromOptionsTest, FailsWithMissingModel) {
|
||||
|
@ -202,24 +199,6 @@ TEST_F(CreateFromOptionsTest, FailsWithMissingModel) {
|
|||
MediaPipeTasksStatus::kRunnerInitializationError))));
|
||||
}
|
||||
|
||||
TEST_F(CreateFromOptionsTest, FailsWithUnspecifiedOutputType) {
|
||||
auto options = std::make_unique<ImageSegmenterOptions>();
|
||||
options->mutable_base_options()->mutable_model_file()->set_file_name(
|
||||
JoinPath("./", kTestDataDirectory, kDeeplabV3WithMetadata));
|
||||
options->mutable_segmenter_options()->set_output_type(
|
||||
SegmenterOptions::UNSPECIFIED);
|
||||
|
||||
auto segmenter_or = ImageSegmenter::Create(
|
||||
std::move(options), absl::make_unique<DeepLabOpResolver>());
|
||||
|
||||
EXPECT_EQ(segmenter_or.status().code(), absl::StatusCode::kInvalidArgument);
|
||||
EXPECT_THAT(segmenter_or.status().message(),
|
||||
HasSubstr("`output_type` must not be UNSPECIFIED"));
|
||||
EXPECT_THAT(segmenter_or.status().GetPayload(kMediaPipeTasksPayload),
|
||||
Optional(absl::Cord(absl::StrCat(
|
||||
MediaPipeTasksStatus::kRunnerInitializationError))));
|
||||
}
|
||||
|
||||
class SegmentationTest : public tflite_shims::testing::Test {};
|
||||
|
||||
TEST_F(SegmentationTest, SucceedsWithCategoryMask) {
|
||||
|
@ -228,10 +207,10 @@ TEST_F(SegmentationTest, SucceedsWithCategoryMask) {
|
|||
DecodeImageFromFile(JoinPath("./", kTestDataDirectory,
|
||||
"segmentation_input_rotation0.jpg")));
|
||||
auto options = std::make_unique<ImageSegmenterOptions>();
|
||||
options->mutable_segmenter_options()->set_output_type(
|
||||
SegmenterOptions::CATEGORY_MASK);
|
||||
options->mutable_base_options()->mutable_model_file()->set_file_name(
|
||||
JoinPath("./", kTestDataDirectory, kDeeplabV3WithMetadata));
|
||||
options->base_options.model_file_name =
|
||||
JoinPath("./", kTestDataDirectory, kDeeplabV3WithMetadata);
|
||||
options->output_type = ImageSegmenterOptions::OutputType::CATEGORY_MASK;
|
||||
|
||||
MP_ASSERT_OK_AND_ASSIGN(std::unique_ptr<ImageSegmenter> segmenter,
|
||||
ImageSegmenter::Create(std::move(options)));
|
||||
MP_ASSERT_OK_AND_ASSIGN(auto category_masks, segmenter->Segment(image));
|
||||
|
@ -253,12 +232,11 @@ TEST_F(SegmentationTest, SucceedsWithConfidenceMask) {
|
|||
Image image,
|
||||
DecodeImageFromFile(JoinPath("./", kTestDataDirectory, "cat.jpg")));
|
||||
auto options = std::make_unique<ImageSegmenterOptions>();
|
||||
options->mutable_segmenter_options()->set_output_type(
|
||||
SegmenterOptions::CONFIDENCE_MASK);
|
||||
options->mutable_segmenter_options()->set_activation(
|
||||
SegmenterOptions::SOFTMAX);
|
||||
options->mutable_base_options()->mutable_model_file()->set_file_name(
|
||||
JoinPath("./", kTestDataDirectory, kDeeplabV3WithMetadata));
|
||||
options->base_options.model_file_name =
|
||||
JoinPath("./", kTestDataDirectory, kDeeplabV3WithMetadata);
|
||||
options->output_type = ImageSegmenterOptions::OutputType::CONFIDENCE_MASK;
|
||||
options->activation = ImageSegmenterOptions::Activation::SOFTMAX;
|
||||
|
||||
MP_ASSERT_OK_AND_ASSIGN(std::unique_ptr<ImageSegmenter> segmenter,
|
||||
ImageSegmenter::Create(std::move(options)));
|
||||
MP_ASSERT_OK_AND_ASSIGN(auto results, segmenter->Segment(image));
|
||||
|
@ -281,17 +259,15 @@ TEST_F(SegmentationTest, SucceedsSelfie128x128Segmentation) {
|
|||
Image image =
|
||||
GetSRGBImage(JoinPath("./", kTestDataDirectory, "mozart_square.jpg"));
|
||||
auto options = std::make_unique<ImageSegmenterOptions>();
|
||||
options->mutable_segmenter_options()->set_output_type(
|
||||
SegmenterOptions::CONFIDENCE_MASK);
|
||||
options->mutable_segmenter_options()->set_activation(
|
||||
SegmenterOptions::SOFTMAX);
|
||||
options->mutable_base_options()->mutable_model_file()->set_file_name(
|
||||
JoinPath("./", kTestDataDirectory, kSelfie128x128WithMetadata));
|
||||
MP_ASSERT_OK_AND_ASSIGN(
|
||||
std::unique_ptr<ImageSegmenter> segmenter,
|
||||
ImageSegmenter::Create(
|
||||
std::move(options),
|
||||
absl::make_unique<SelfieSegmentationModelOpResolver>()));
|
||||
options->base_options.model_file_name =
|
||||
JoinPath("./", kTestDataDirectory, kSelfie128x128WithMetadata);
|
||||
options->base_options.op_resolver =
|
||||
absl::make_unique<SelfieSegmentationModelOpResolver>();
|
||||
options->output_type = ImageSegmenterOptions::OutputType::CONFIDENCE_MASK;
|
||||
options->activation = ImageSegmenterOptions::Activation::SOFTMAX;
|
||||
|
||||
MP_ASSERT_OK_AND_ASSIGN(std::unique_ptr<ImageSegmenter> segmenter,
|
||||
ImageSegmenter::Create(std::move(options)));
|
||||
MP_ASSERT_OK_AND_ASSIGN(auto confidence_masks, segmenter->Segment(image));
|
||||
EXPECT_EQ(confidence_masks.size(), 2);
|
||||
|
||||
|
@ -313,15 +289,14 @@ TEST_F(SegmentationTest, SucceedsSelfie144x256Segmentations) {
|
|||
Image image =
|
||||
GetSRGBImage(JoinPath("./", kTestDataDirectory, "mozart_square.jpg"));
|
||||
auto options = std::make_unique<ImageSegmenterOptions>();
|
||||
options->mutable_segmenter_options()->set_output_type(
|
||||
SegmenterOptions::CONFIDENCE_MASK);
|
||||
options->mutable_base_options()->mutable_model_file()->set_file_name(
|
||||
JoinPath("./", kTestDataDirectory, kSelfie144x256WithMetadata));
|
||||
MP_ASSERT_OK_AND_ASSIGN(
|
||||
std::unique_ptr<ImageSegmenter> segmenter,
|
||||
ImageSegmenter::Create(
|
||||
std::move(options),
|
||||
absl::make_unique<SelfieSegmentationModelOpResolver>()));
|
||||
options->base_options.model_file_name =
|
||||
JoinPath("./", kTestDataDirectory, kSelfie144x256WithMetadata);
|
||||
options->base_options.op_resolver =
|
||||
absl::make_unique<SelfieSegmentationModelOpResolver>();
|
||||
options->output_type = ImageSegmenterOptions::OutputType::CONFIDENCE_MASK;
|
||||
options->activation = ImageSegmenterOptions::Activation::NONE;
|
||||
MP_ASSERT_OK_AND_ASSIGN(std::unique_ptr<ImageSegmenter> segmenter,
|
||||
ImageSegmenter::Create(std::move(options)));
|
||||
MP_ASSERT_OK_AND_ASSIGN(auto confidence_masks, segmenter->Segment(image));
|
||||
EXPECT_EQ(confidence_masks.size(), 1);
|
||||
|
30
mediapipe/tasks/cc/vision/image_segmenter/proto/BUILD
Normal file
30
mediapipe/tasks/cc/vision/image_segmenter/proto/BUILD
Normal file
|
@ -0,0 +1,30 @@
|
|||
# Copyright 2022 The MediaPipe Authors. All Rights Reserved.
|
||||
#
|
||||
# Licensed under the Apache License, Version 2.0 (the "License");
|
||||
# you may not use this file except in compliance with the License.
|
||||
# You may obtain a copy of the License at
|
||||
#
|
||||
# http://www.apache.org/licenses/LICENSE-2.0
|
||||
#
|
||||
# Unless required by applicable law or agreed to in writing, software
|
||||
# distributed under the License is distributed on an "AS IS" BASIS,
|
||||
# WITHOUT WARRANTIES OR CONDITIONS OF ANY KIND, either express or implied.
|
||||
# See the License for the specific language governing permissions and
|
||||
# limitations under the License.
|
||||
|
||||
load("//mediapipe/framework/port:build_config.bzl", "mediapipe_proto_library")
|
||||
|
||||
package(default_visibility = ["//mediapipe/tasks:internal"])
|
||||
|
||||
licenses(["notice"])
|
||||
|
||||
mediapipe_proto_library(
|
||||
name = "image_segmenter_options_proto",
|
||||
srcs = ["image_segmenter_options.proto"],
|
||||
deps = [
|
||||
"//mediapipe/framework:calculator_options_proto",
|
||||
"//mediapipe/framework:calculator_proto",
|
||||
"//mediapipe/tasks/cc/components:segmenter_options_proto",
|
||||
"//mediapipe/tasks/cc/core/proto:base_options_proto",
|
||||
],
|
||||
)
|
|
@ -15,7 +15,7 @@ limitations under the License.
|
|||
|
||||
syntax = "proto2";
|
||||
|
||||
package mediapipe.tasks;
|
||||
package mediapipe.tasks.vision.image_segmenter.proto;
|
||||
|
||||
import "mediapipe/framework/calculator.proto";
|
||||
import "mediapipe/tasks/cc/components/segmenter_options.proto";
|
||||
|
@ -25,7 +25,7 @@ message ImageSegmenterOptions {
|
|||
extend mediapipe.CalculatorOptions {
|
||||
optional ImageSegmenterOptions ext = 458105758;
|
||||
}
|
||||
// Base options for configuring Task library, such as specifying the TfLite
|
||||
// Base options for configuring MediaPipe Tasks, such as specifying the TfLite
|
||||
// model file with metadata, accelerator options, etc.
|
||||
optional core.proto.BaseOptions base_options = 1;
|
||||
|
|
@ -36,10 +36,19 @@ namespace vision {
|
|||
|
||||
// The options for configuring a mediapipe object detector task.
|
||||
struct ObjectDetectorOptions {
|
||||
// Base options for configuring Task library, such as specifying the TfLite
|
||||
// Base options for configuring MediaPipe Tasks, such as specifying the TfLite
|
||||
// model file with metadata, accelerator options, op resolver, etc.
|
||||
tasks::core::BaseOptions base_options;
|
||||
|
||||
// The running mode of the task. Default to the image mode.
|
||||
// Object detector has three running modes:
|
||||
// 1) The image mode for detecting objects on single image inputs.
|
||||
// 2) The video mode for detecting objects on the decoded frames of a video.
|
||||
// 3) The live stream mode for detecting objects on the live stream of input
|
||||
// data, such as from camera. In this mode, the "result_callback" below must
|
||||
// be specified to receive the detection results asynchronously.
|
||||
core::RunningMode running_mode = core::RunningMode::IMAGE;
|
||||
|
||||
// The locale to use for display names specified through the TFLite Model
|
||||
// Metadata, if any. Defaults to English.
|
||||
std::string display_names_locale = "en";
|
||||
|
@ -65,15 +74,6 @@ struct ObjectDetectorOptions {
|
|||
// category names are ignored. Mutually exclusive with category_allowlist.
|
||||
std::vector<std::string> category_denylist = {};
|
||||
|
||||
// The running mode of the task. Default to the image mode.
|
||||
// Object detector has three running modes:
|
||||
// 1) The image mode for detecting objects on single image inputs.
|
||||
// 2) The video mode for detecting objects on the decoded frames of a video.
|
||||
// 3) The live stream mode for detecting objects on the live stream of input
|
||||
// data, such as from camera. In this mode, the "result_callback" below must
|
||||
// be specified to receive the detection results asynchronously.
|
||||
core::RunningMode running_mode = core::RunningMode::IMAGE;
|
||||
|
||||
// The user-defined result callback for processing live stream data.
|
||||
// The result callback should only be specified when the running mode is set
|
||||
// to RunningMode::LIVE_STREAM.
|
||||
|
|
|
@ -531,9 +531,9 @@ class ObjectDetectorGraph : public core::ModelTaskGraph {
|
|||
// Outputs the labeled detections and the processed image as the subgraph
|
||||
// output streams.
|
||||
return {{
|
||||
.detections =
|
||||
detection_label_id_to_text[Output<std::vector<Detection>>("")],
|
||||
.image = preprocessing[Output<Image>(kImageTag)],
|
||||
/* detections= */
|
||||
detection_label_id_to_text[Output<std::vector<Detection>>("")],
|
||||
/* image= */ preprocessing[Output<Image>(kImageTag)],
|
||||
}};
|
||||
}
|
||||
};
|
||||
|
|
|
@ -194,7 +194,7 @@ TEST_F(CreateFromOptionsTest, FailsWithSelectiveOpResolverMissingOps) {
|
|||
// interpreter errors (e.g., "Encountered unresolved custom op").
|
||||
EXPECT_EQ(object_detector.status().code(), absl::StatusCode::kInternal);
|
||||
EXPECT_THAT(object_detector.status().message(),
|
||||
HasSubstr("interpreter_->AllocateTensors() == kTfLiteOk"));
|
||||
HasSubstr("interpreter->AllocateTensors() == kTfLiteOk"));
|
||||
}
|
||||
|
||||
TEST_F(CreateFromOptionsTest, FailsWithMissingModel) {
|
||||
|
|
|
@ -27,7 +27,7 @@ message ObjectDetectorOptions {
|
|||
extend mediapipe.CalculatorOptions {
|
||||
optional ObjectDetectorOptions ext = 443442058;
|
||||
}
|
||||
// Base options for configuring Task library, such as specifying the TfLite
|
||||
// Base options for configuring MediaPipe Tasks, such as specifying the TfLite
|
||||
// model file with metadata, accelerator options, etc.
|
||||
optional core.proto.BaseOptions base_options = 1;
|
||||
|
||||
|
|
|
@ -1,75 +0,0 @@
|
|||
/* Copyright 2022 The MediaPipe Authors. All Rights Reserved.
|
||||
|
||||
Licensed under the Apache License, Version 2.0 (the "License");
|
||||
you may not use this file except in compliance with the License.
|
||||
You may obtain a copy of the License at
|
||||
|
||||
http://www.apache.org/licenses/LICENSE-2.0
|
||||
|
||||
Unless required by applicable law or agreed to in writing, software
|
||||
distributed under the License is distributed on an "AS IS" BASIS,
|
||||
WITHOUT WARRANTIES OR CONDITIONS OF ANY KIND, either express or implied.
|
||||
See the License for the specific language governing permissions and
|
||||
limitations under the License.
|
||||
==============================================================================*/
|
||||
|
||||
#include "mediapipe/tasks/cc/vision/segmentation/image_segmenter.h"
|
||||
|
||||
#include "mediapipe/framework/api2/builder.h"
|
||||
#include "mediapipe/tasks/cc/core/task_api_factory.h"
|
||||
|
||||
namespace mediapipe {
|
||||
namespace tasks {
|
||||
namespace vision {
|
||||
namespace {
|
||||
|
||||
constexpr char kSegmentationStreamName[] = "segmented_mask_out";
|
||||
constexpr char kGroupedSegmentationTag[] = "GROUPED_SEGMENTATION";
|
||||
constexpr char kImageStreamName[] = "image_in";
|
||||
constexpr char kImageTag[] = "IMAGE";
|
||||
constexpr char kSubgraphTypeName[] =
|
||||
"mediapipe.tasks.vision.ImageSegmenterGraph";
|
||||
|
||||
using ::mediapipe::CalculatorGraphConfig;
|
||||
using ::mediapipe::Image;
|
||||
|
||||
// Creates a MediaPipe graph config that only contains a single subgraph node of
|
||||
// "mediapipe.tasks.vision.SegmenterGraph".
|
||||
CalculatorGraphConfig CreateGraphConfig(
|
||||
std::unique_ptr<ImageSegmenterOptions> options) {
|
||||
api2::builder::Graph graph;
|
||||
auto& subgraph = graph.AddNode(kSubgraphTypeName);
|
||||
subgraph.GetOptions<ImageSegmenterOptions>().Swap(options.get());
|
||||
graph.In(kImageTag).SetName(kImageStreamName) >> subgraph.In(kImageTag);
|
||||
subgraph.Out(kGroupedSegmentationTag).SetName(kSegmentationStreamName) >>
|
||||
graph.Out(kGroupedSegmentationTag);
|
||||
return graph.GetConfig();
|
||||
}
|
||||
|
||||
} // namespace
|
||||
|
||||
absl::StatusOr<std::unique_ptr<ImageSegmenter>> ImageSegmenter::Create(
|
||||
std::unique_ptr<ImageSegmenterOptions> options,
|
||||
std::unique_ptr<tflite::OpResolver> resolver) {
|
||||
return core::TaskApiFactory::Create<ImageSegmenter, ImageSegmenterOptions>(
|
||||
CreateGraphConfig(std::move(options)), std::move(resolver));
|
||||
}
|
||||
|
||||
absl::StatusOr<std::vector<Image>> ImageSegmenter::Segment(
|
||||
mediapipe::Image image) {
|
||||
if (image.UsesGpu()) {
|
||||
return CreateStatusWithPayload(
|
||||
absl::StatusCode::kInvalidArgument,
|
||||
absl::StrCat("GPU input images are currently not supported."),
|
||||
MediaPipeTasksStatus::kRunnerUnexpectedInputError);
|
||||
}
|
||||
ASSIGN_OR_RETURN(
|
||||
auto output_packets,
|
||||
runner_->Process({{kImageStreamName,
|
||||
mediapipe::MakePacket<Image>(std::move(image))}}));
|
||||
return output_packets[kSegmentationStreamName].Get<std::vector<Image>>();
|
||||
}
|
||||
|
||||
} // namespace vision
|
||||
} // namespace tasks
|
||||
} // namespace mediapipe
|
|
@ -1,76 +0,0 @@
|
|||
/* Copyright 2022 The MediaPipe Authors. All Rights Reserved.
|
||||
|
||||
Licensed under the Apache License, Version 2.0 (the "License");
|
||||
you may not use this file except in compliance with the License.
|
||||
You may obtain a copy of the License at
|
||||
|
||||
http://www.apache.org/licenses/LICENSE-2.0
|
||||
|
||||
Unless required by applicable law or agreed to in writing, software
|
||||
distributed under the License is distributed on an "AS IS" BASIS,
|
||||
WITHOUT WARRANTIES OR CONDITIONS OF ANY KIND, either express or implied.
|
||||
See the License for the specific language governing permissions and
|
||||
limitations under the License.
|
||||
==============================================================================*/
|
||||
|
||||
#ifndef MEDIAPIPE_TASKS_CC_VISION_SEGMENTATION_IMAGE_SEGMENTER_H_
|
||||
#define MEDIAPIPE_TASKS_CC_VISION_SEGMENTATION_IMAGE_SEGMENTER_H_
|
||||
|
||||
#include <memory>
|
||||
#include <utility>
|
||||
#include <vector>
|
||||
|
||||
#include "absl/memory/memory.h"
|
||||
#include "absl/status/statusor.h"
|
||||
#include "mediapipe/framework/formats/image.h"
|
||||
#include "mediapipe/tasks/cc/core/base_task_api.h"
|
||||
#include "mediapipe/tasks/cc/vision/segmentation/image_segmenter_options.pb.h"
|
||||
#include "tensorflow/lite/core/api/op_resolver.h"
|
||||
#include "tensorflow/lite/kernels/register.h"
|
||||
|
||||
namespace mediapipe {
|
||||
namespace tasks {
|
||||
namespace vision {
|
||||
|
||||
// Performs segmentation on images.
|
||||
//
|
||||
// The API expects a TFLite model with mandatory TFLite Model Metadata.
|
||||
//
|
||||
// Input tensor:
|
||||
// (kTfLiteUInt8/kTfLiteFloat32)
|
||||
// - image input of size `[batch x height x width x channels]`.
|
||||
// - batch inference is not supported (`batch` is required to be 1).
|
||||
// - RGB and greyscale inputs are supported (`channels` is required to be
|
||||
// 1 or 3).
|
||||
// - if type is kTfLiteFloat32, NormalizationOptions are required to be
|
||||
// attached to the metadata for input normalization.
|
||||
// Output tensors:
|
||||
// (kTfLiteUInt8/kTfLiteFloat32)
|
||||
// - list of segmented masks.
|
||||
// - if `output_type` is CATEGORY_MASK, uint8 Image, Image vector of size 1.
|
||||
// - if `output_type` is CONFIDENCE_MASK, float32 Image list of size
|
||||
// `cahnnels`.
|
||||
// - batch is always 1
|
||||
// An example of such model can be found at:
|
||||
// https://tfhub.dev/tensorflow/lite-model/deeplabv3/1/metadata/2
|
||||
class ImageSegmenter : core::BaseTaskApi {
|
||||
public:
|
||||
using BaseTaskApi::BaseTaskApi;
|
||||
|
||||
// Creates a Segmenter from the provided options. A non-default
|
||||
// OpResolver can be specified in order to support custom Ops or specify a
|
||||
// subset of built-in Ops.
|
||||
static absl::StatusOr<std::unique_ptr<ImageSegmenter>> Create(
|
||||
std::unique_ptr<ImageSegmenterOptions> options,
|
||||
std::unique_ptr<tflite::OpResolver> resolver =
|
||||
absl::make_unique<tflite::ops::builtin::BuiltinOpResolver>());
|
||||
|
||||
// Runs the actual segmentation task.
|
||||
absl::StatusOr<std::vector<mediapipe::Image>> Segment(mediapipe::Image image);
|
||||
};
|
||||
|
||||
} // namespace vision
|
||||
} // namespace tasks
|
||||
} // namespace mediapipe
|
||||
|
||||
#endif // MEDIAPIPE_TASKS_CC_VISION_SEGMENTATION_IMAGE_SEGMENTER_H_
|
1
mediapipe/tasks/testdata/vision/BUILD
vendored
1
mediapipe/tasks/testdata/vision/BUILD
vendored
|
@ -80,6 +80,7 @@ filegroup(
|
|||
],
|
||||
)
|
||||
|
||||
# TODO Create individual filegroup for models required for each Tasks.
|
||||
filegroup(
|
||||
name = "test_models",
|
||||
srcs = [
|
||||
|
|
Loading…
Reference in New Issue
Block a user